{ "cells": [ { "cell_type": "code", "execution_count": 1, "metadata": { "slideshow": { "slide_type": "skip" } }, "outputs": [ { "data": { "text/html": [ "<script>\n", " function code_toggle() {\n", " if (code_shown){\n", " $('div.input').hide('500');\n", " $('#toggleButton').val('Show Code')\n", " } else {\n", " $('div.input').show('500');\n", " $('#toggleButton').val('Hide Code')\n", " }\n", " code_shown = !code_shown\n", " }\n", "\n", " $( document ).ready(function(){\n", " code_shown=false;\n", " $('div.input').hide()\n", " });\n", "</script>\n", "<form action=\"javascript:code_toggle()\"><input type=\"submit\" id=\"toggleButton\" value=\"Show Code\"></form>\n", "<style>\n", ".rendered_html td {\n", " font-size: xx-large;\n", " text-align: left; !important\n", "}\n", ".rendered_html th {\n", " font-size: xx-large;\n", " text-align: left; !important\n", "}\n", "</style>" ], "text/plain": [ "<IPython.core.display.HTML object>" ] }, "metadata": {}, "output_type": "display_data" } ], "source": [ "%%html\n", "<script>\n", " function code_toggle() {\n", " if (code_shown){\n", " $('div.input').hide('500');\n", " $('#toggleButton').val('Show Code')\n", " } else {\n", " $('div.input').show('500');\n", " $('#toggleButton').val('Hide Code')\n", " }\n", " code_shown = !code_shown\n", " }\n", "\n", " $( document ).ready(function(){\n", " code_shown=false;\n", " $('div.input').hide()\n", " });\n", "</script>\n", "<form action=\"javascript:code_toggle()\"><input type=\"submit\" id=\"toggleButton\" value=\"Show Code\"></form>\n", "<style>\n", ".rendered_html td {\n", " font-size: xx-large;\n", " text-align: left; !important\n", "}\n", ".rendered_html th {\n", " font-size: xx-large;\n", " text-align: left; !important\n", "}\n", "</style>" ] }, { "cell_type": "code", "execution_count": 2, "metadata": { "slideshow": { "slide_type": "skip" } }, "outputs": [], "source": [ "%%capture\n", "%load_ext autoreload\n", "%autoreload 2\n", "import sys\n", "sys.path.append(\"../statnlpbook/\")\n", "\n", "#util.execute_notebook('relation_extraction.ipynb')" ] }, { "cell_type": "markdown", "metadata": { "slideshow": { "slide_type": "skip" } }, "source": [ "<!---\n", "Latex Macros\n", "-->\n", "$$\n", "\\newcommand{\\Xs}{\\mathcal{X}}\n", "\\newcommand{\\Ys}{\\mathcal{Y}}\n", "\\newcommand{\\y}{\\mathbf{y}}\n", "\\newcommand{\\balpha}{\\boldsymbol{\\alpha}}\n", "\\newcommand{\\bbeta}{\\boldsymbol{\\beta}}\n", "\\newcommand{\\aligns}{\\mathbf{a}}\n", "\\newcommand{\\align}{a}\n", "\\newcommand{\\source}{\\mathbf{s}}\n", "\\newcommand{\\target}{\\mathbf{t}}\n", "\\newcommand{\\ssource}{s}\n", "\\newcommand{\\starget}{t}\n", "\\newcommand{\\repr}{\\mathbf{f}}\n", "\\newcommand{\\repry}{\\mathbf{g}}\n", "\\newcommand{\\x}{\\mathbf{x}}\n", "\\newcommand{\\prob}{p}\n", "\\newcommand{\\a}{\\alpha}\n", "\\newcommand{\\b}{\\beta}\n", "\\newcommand{\\vocab}{V}\n", "\\newcommand{\\params}{\\boldsymbol{\\theta}}\n", "\\newcommand{\\param}{\\theta}\n", "\\DeclareMathOperator{\\perplexity}{PP}\n", "\\DeclareMathOperator{\\argmax}{argmax}\n", "\\DeclareMathOperator{\\argmin}{argmin}\n", "\\newcommand{\\train}{\\mathcal{D}}\n", "\\newcommand{\\counts}[2]{\\#_{#1}(#2) }\n", "\\newcommand{\\length}[1]{\\text{length}(#1) }\n", "\\newcommand{\\indi}{\\mathbb{I}}\n", "$$" ] }, { "cell_type": "code", "execution_count": 3, "metadata": { "slideshow": { "slide_type": "skip" } }, "outputs": [], "source": [ "%load_ext tikzmagic" ] }, { "cell_type": "markdown", "metadata": { "slideshow": { "slide_type": "slide" } }, "source": [ "<img src=\"https://imgs.xkcd.com/comics/easy_or_hard.png\" width=80%/>" ] }, { "cell_type": "markdown", "metadata": { "slideshow": { "slide_type": "slide" } }, "source": [ "# Question Answering" ] }, { "cell_type": "markdown", "metadata": { "slideshow": { "slide_type": "fragment" } }, "source": [ "* Flavours of question answering\n", "* Information retrieval\n", "* Machine reading comprehension\n", "* Question answering from structured knowledge\n", "* Executable semantic parsing\n", "* Relation extraction via reading comprehension" ] }, { "cell_type": "markdown", "metadata": { "slideshow": { "slide_type": "slide" } }, "source": [ "Question:\n", "\n", ">Which university did Turing go to?" ] }, { "cell_type": "markdown", "metadata": { "slideshow": { "slide_type": "fragment" } }, "source": [ "Answer:\n", "\n", ">Princeton" ] }, { "cell_type": "markdown", "metadata": { "slideshow": { "slide_type": "fragment" } }, "source": [ "[Passage](https://en.wikipedia.org/wiki/Alan_Turing):\n", "\n", "> In 1938, he obtained his PhD from the Department of Mathematics at Princeton University." ] }, { "cell_type": "markdown", "metadata": { "slideshow": { "slide_type": "fragment" } }, "source": [ "Knowledge base:\n", "\n", "https://www.wikidata.org/wiki/Q7251#P69" ] }, { "cell_type": "markdown", "metadata": { "slideshow": { "slide_type": "subslide" } }, "source": [ "<center><img src=\"../img/quiz_time.png\"></center>\n", "\n", "## [ucph.page.link/qa](https://ucph.page.link/qa)\n", "\n", "([Responses](https://docs.google.com/forms/d/17j4Msqa_L_so14KGZEdGClZaAbRsvn0rQedeze8kaRM/edit#responses))" ] }, { "cell_type": "markdown", "metadata": { "slideshow": { "slide_type": "slide" } }, "source": [ "## Flavours of Question answering (QA)\n", "\n", "Factoid questions:\n", "\n", "* Information retrieval (IR)-based QA on **unstructured** data (text)\n", "* Knowledge-based QA on **structured** data (DB/KB)\n", "\n", "Non-factoid questions:\n", "\n", "* \"How\" questions\n", "> How do I delete my Instagram account?\n", "* \"Why\" questions\n", "> Why is the sky blue?" ] }, { "cell_type": "markdown", "metadata": { "slideshow": { "slide_type": "skip" } }, "source": [ "* Math problems\n", "<img src=\"../img/geometry.png\" width=50%/>\n", "<div style=\"text-align: right;\">\n", " (from <a href=\"https://aclanthology.org/2021.acl-long.528/\">Lu et al., 2021</a>)\n", "</div>" ] }, { "cell_type": "markdown", "metadata": { "slideshow": { "slide_type": "subslide" } }, "source": [ "### Formats\n", "\n", "* Extractive (span selection)\n", "* Cloze\n", "* Boolean\n", "* Multi-choice\n", "* Abstractive" ] }, { "cell_type": "markdown", "metadata": { "slideshow": { "slide_type": "subslide" } }, "source": [ "### QA on collections of documents\n", "\n", "* [TriviaQA](https://nlp.cs.washington.edu/triviaqa/)\n", "* [SearchQA](https://github.com/nyu-dl/dl4ir-searchqA)\n", "* [MS MARCO](https://microsoft.github.io/msmarco/)\n", "* [AmazonQA](https://github.com/amazonqa/amazonqa)\n", "* [TrecQA](https://trec.nist.gov/data/qa.html)\n", "* [WebQA](https://webqna.github.io/)\n", "\n", "> Which politician won the Nobel Peace Prize in 2009?" ] }, { "cell_type": "markdown", "metadata": { "slideshow": { "slide_type": "subslide" } }, "source": [ "### Multi-hop QA\n", "\n", "* [HotPotQA](https://hotpotqa.github.io/)\n", "* [QAngaroo](https://qangaroo.cs.ucl.ac.uk/)\n", "* [ComplexWebQuestions](https://www.tau-nlp.sites.tau.ac.il/compwebq)\n", "* [HybridQA](https://hybridqa.github.io/)\n", "\n", "> What is the middle name of the player with the second most National Football League career rushing yards?" ] }, { "cell_type": "markdown", "metadata": { "slideshow": { "slide_type": "subslide" } }, "source": [ "### QA on structured knowledge\n", "\n", "* [FreebaseQA](https://github.com/kelvin-jiang/FreebaseQA)\n", "* [Event-QA](https://github.com/tarcisiosouza/Event-QA)\n", "* [WikiTableQuestions](https://github.com/ppasupat/WikiTableQuestions)\n", "* [SimpleQuestions](https://github.com/davidgolub/SimpleQA)\n", "* [WikiSQL](https://github.com/salesforce/WikiSQL)\n", "* [RuBQ](https://github.com/vladislavneon/RuBQ)\n", "\n", "> The 1999 film '10 Things I Hate About You' is based on which Shakespeare play?" ] }, { "cell_type": "markdown", "metadata": { "slideshow": { "slide_type": "subslide" } }, "source": [ "### Extractive QA\n", "\n", "* [SQuAD](https://rajpurkar.github.io/SQuAD-explorer/)\n", "* [Natural Questions](https://ai.google.com/research/NaturalQuestions/)\n", "* [NewsQA](https://www.microsoft.com/en-us/research/project/newsqa-dataset/)\n", "* [TyDI-QA](https://github.com/google-research-datasets/tydiqa)\n", "\n", "<center>\n", " <img src=\"https://rajpurkar.github.io/mlx/qa-and-squad/example-squad.png\" width=\"70%\">\n", "</center>\n", "\n", "<div style=\"text-align: right;\">\n", " (from <a href=\"https://www.aclweb.org/anthology/D16-1264\">Rajpurkar et al., 2016</a>)\n", "</div>" ] }, { "cell_type": "markdown", "metadata": { "slideshow": { "slide_type": "slide" } }, "source": [ "## Information retrieval (IR)-based QA\n", "\n", "General approach:\n", "1. Retrieve relevant **passage**(s)\n", "2. Machine reading comprehension: extract the **answer**, which can be\n", " * A text span from the passage\n", " * Yes/no\n", " * `NULL` (unanswerable)\n" ] }, { "cell_type": "markdown", "metadata": { "slideshow": { "slide_type": "subslide" } }, "source": [ "### Information retrieval\n", "\n", "* Obtain relevant documents/passages given a query\n", "* Example: web search\n", "* Learn more in [NDAK20002U Neural Information Retrieval (NIR)](https://kurser.ku.dk/course/ndak20002u/)" ] }, { "cell_type": "markdown", "metadata": { "slideshow": { "slide_type": "subslide" } }, "source": [ "### Machine reading comprehension (MRC)\n", "\n", "* Input: (Passage, Question)\n", "* Output: Answer span\n", "\n", "<center>\n", " <img src=\"qa_figures/squad.png\" width=\"38%\">\n", "</center>\n", "\n", "<div style=\"text-align: right;\">\n", " (from <a href=\"https://www.aclweb.org/anthology/D16-1264\">Rajpurkar et al., 2016</a>)\n", "</div>" ] }, { "cell_type": "markdown", "metadata": { "slideshow": { "slide_type": "fragment" } }, "source": [ "### [demo](https://demo.allennlp.org/reading-comprehension/transformer-qa)" ] }, { "cell_type": "markdown", "metadata": { "slideshow": { "slide_type": "subslide" } }, "source": [ "### MRC modeling\n", "\n", "How to model span selection?\n", "\n", "* As sequence labeling (for each token, is it part of the answer?)\n", " * With IOB encoding\n", " \n", "<center>\n", " <img src=\"../img/slqa.jpeg\" width=50%/>\n", "</center>\n", "<div style=\"text-align: right\">\n", " (from <a href=\"https://doi.org/10.1093/bioinformatics/btac397\">Yoon et al., 2022</a>)\n", "</div>" ] }, { "cell_type": "markdown", "metadata": { "slideshow": { "slide_type": "fragment" } }, "source": [ "* Or as span selection (find start and end of the answer span)\n", "\n", "||||start|end||||\n", "|---|---|---|---|---|---|---|---|\n", "|...|termed|the|interferon|signature|.|Here|...|" ] }, { "cell_type": "markdown", "metadata": { "slideshow": { "slide_type": "fragment" } }, "source": [ "What are the advantages and disadvantages of each?" ] }, { "cell_type": "markdown", "metadata": { "slideshow": { "slide_type": "subslide" } }, "source": [ "### MRC evaluation\n", "\n", "Metrics for binary (yes/no) QA:\n", "* **Accuracy**\n", "* **F-score**" ] }, { "cell_type": "markdown", "metadata": { "slideshow": { "slide_type": "skip" } }, "source": [ "Metrics for ranking:\n", "* **MRR** (mean reciprocal rank)" ] }, { "cell_type": "markdown", "metadata": { "slideshow": { "slide_type": "fragment" } }, "source": [ "Test questions have $k$ gold answers by different human annotators (e.g., $k=3$ for SQuAD and TyDI-QA, $k=5$ for NQ).\n", "Metrics for span selection:\n", "* **Exact match** (EM): text is exactly any of the $k$\n", "* **Word-based f-score** (like in some [sequence labeling](sequence_labeling_slides.ipynb) tasks) averaged over the $k$ gold answers\n", " * Often ignoring punctuation and articles, i.e., `a, an, the`\n", " * As bag-of-words, not exact positions (because the same answer may appear multiple times)\n", " * Macro-averaged: calculate f-score for each question and average the f-scores" ] }, { "cell_type": "markdown", "metadata": { "slideshow": { "slide_type": "subslide" } }, "source": [ "### SQuAD\n", "\n", "<center>\n", " <a href=\"slides/cs224n-2020-lecture10-QA.pdf\"><img src=\"../img/squad_leaderboard.png\" width=37%></a>\n", "</center>\n", "\n", "<div style=\"text-align: right;\">\n", " (from <a href=\"https://rajpurkar.github.io/SQuAD-explorer/\">SQuAD leaderboard</a>)\n", "</div>" ] }, { "cell_type": "markdown", "metadata": { "slideshow": { "slide_type": "skip" } }, "source": [ "### MRC Models\n", "\n", "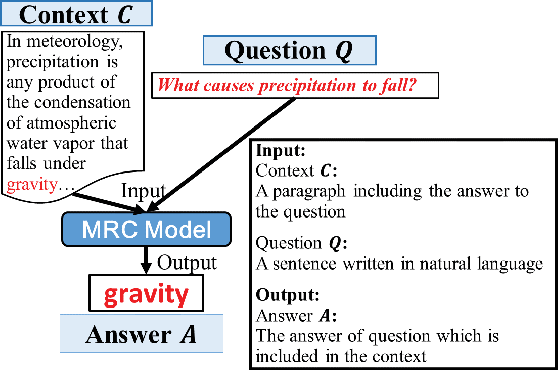\n", "\n", "<div style=\"text-align: right;\">\n", " (from <a href=\"https://aclanthology.org/2020.aacl-srw.21/\">Li et al., 2020</a>)\n", "</div>" ] }, { "cell_type": "markdown", "metadata": { "slideshow": { "slide_type": "subslide" } }, "source": [ "### MRC with BERT\n", "\n", "<center>\n", " <img src=\"../img/bert_qa.png\" width=50%/>\n", "</center>\n", "\n", "<div style=\"text-align: right;\">\n", " (from <a href=\"https://www.aclweb.org/anthology/N19-1423.pdf\">Devlin et al., 2019</a>)\n", "</div>" ] }, { "cell_type": "markdown", "metadata": { "slideshow": { "slide_type": "subslide" } }, "source": [ "### SpanBERT\n", "\n", "<img src=\"../img/SpanBERT.png\" width=100%/>\n", "\n", "(from [Joshi et al., 2020](https://aclanthology.org/2020.tacl-1.5))" ] }, { "cell_type": "markdown", "metadata": { "slideshow": { "slide_type": "subslide" } }, "source": [ "### MRC skills\n", "\n", "<img src=\"https://d3i71xaburhd42.cloudfront.net/0e6e8274d0dcbc1c3c1ccdbd87f3e5d53fdf62b4/19-Figure3-1.png\" width=100%/>\n", "\n", "(from [Rogers et al., 2022](https://dl.acm.org/doi/10.1145/3560260))" ] }, { "cell_type": "markdown", "metadata": { "slideshow": { "slide_type": "slide" } }, "source": [ "## Knowledge-based (KB) question answering\n", "\n", "Information is already organized in tables, databases and knowledge bases!\n", "\n", "1. (Executable) **semantic parsing**: translate natural language question to SQL/SPARQL/logical form **program** (query).\n", "2. **Execute** the program on a database/knowledge-base and return the answer." ] }, { "cell_type": "markdown", "metadata": { "slideshow": { "slide_type": "fragment" } }, "source": [ "\n", "\n", "[Which university did Turing go to?](https://query.wikidata.org/#select%20distinct%20%3Fitem%20%3FitemLabel%20where%20%7B%0A%20%20%20%20%3Fitem%20wdt%3AP31%20wd%3AQ15936437.%0A%20%20%20%20wd%3AQ7251%20wdt%3AP69%20%3Fitem.%0A%20%20%20%20SERVICE%20wikibase%3Alabel%20%7B%20bd%3AserviceParam%20wikibase%3Alanguage%20%22en%22%20%7D%0A%7D%0AORDER%20BY%20DESC%28%3Fsitelinks%29)" ] }, { "cell_type": "markdown", "metadata": { "slideshow": { "slide_type": "subslide" } }, "source": [ "<img src=\"../img/mcwq.png\" width=90%/>\n", "\n", "(from [Cui et al., 2022](https://aclanthology.org/2022.tacl-1.55/))" ] }, { "cell_type": "markdown", "metadata": { "slideshow": { "slide_type": "subslide" } }, "source": [ "### Semantic parsing as machine translation\n", "\n", "Can be modeled as sequence-to-sequence (seq2seq), see [machine translation](nmt_slides_active.ipynb).\n", "\n", "<img src=\"https://guide.allennlp.org/part3/semantic-parsing/database-interface.svg\" width=80%/>\n", "\n", "(from [AllenNLP](https://guide.allennlp.org/semantic-parsing-seq2seq))" ] }, { "cell_type": "markdown", "metadata": { "slideshow": { "slide_type": "skip" } }, "source": [ "### Executable semantic parsing to SPARQL\n", "\n", "<center>\n", " <img src=\"https://d3i71xaburhd42.cloudfront.net/8b65582bcb84b30393c67a2bae71a9e84f45e87c/4-Figure1-1.png\" width=\"100%\">\n", "</center>\n", "\n", "<div style=\"text-align: right;\">\n", " (from <a href=\"https://arxiv.org/pdf/1912.09713.pdf\">Keysers et al., 2020</a>)\n", "</div>" ] }, { "cell_type": "markdown", "metadata": { "slideshow": { "slide_type": "skip" } }, "source": [ "### Executable semantic parsing to SQL\n", "\n", "<center>\n", " <img src=\"https://d3i71xaburhd42.cloudfront.net/37882abaec01eba1bf5bda8a36c904aaea0d5642/6-Table1-1.png\" width=\"80%\">\n", "</center>\n", "\n", "<div style=\"text-align: right;\">\n", " (from <a href=\"https://arxiv.org/pdf/2010.05647.pdf\">Oren et al., 2020</a>)\n", "</div>" ] }, { "cell_type": "markdown", "metadata": { "slideshow": { "slide_type": "skip" } }, "source": [ "### Executable semantic parsing to SQL\n", "\n", "<center>\n", " <img src=\"https://d3i71xaburhd42.cloudfront.net/23474a845ea4b67f38bde7c7f1c4c1bdba22c50c/1-Figure1-1.png\" width=\"80%\">\n", "</center>\n", "\n", "<div style=\"text-align: right;\">\n", " (from <a href=\"https://www.aclweb.org/anthology/P18-1033\">Finegan-Dollak et al., 2018</a>)\n", "</div>" ] }, { "cell_type": "markdown", "metadata": { "slideshow": { "slide_type": "skip" } }, "source": [ "### Executable semantic parsing to logical form\n", "\n", "<center>\n", " <img src=\"https://d3i71xaburhd42.cloudfront.net/b29447ba499507a259ae9d8f685d60cc1597d7d3/1-Figure1-1.png\" width=\"50%\">\n", "</center>\n", "\n", "<div style=\"text-align: right;\">\n", " (from <a href=\"https://www.aclweb.org/anthology/D13-1160.pdf\">Berant et al., 2013</a>)\n", "</div>" ] }, { "cell_type": "markdown", "metadata": { "slideshow": { "slide_type": "slide" } }, "source": [ "## Relation extraction via reading comprehension\n", "\n", " \n", "\n", "<center>\n", " <a href=\"slides/zeroshot-relation-extraction-via-reading-comprehension-conll-2017.pdf\">\n", " <img src=\"https://d3i71xaburhd42.cloudfront.net/fa025e5d117929361bcf798437957762eb5bb6d4/4-Figure2-1.png\" width=\"100%\">\n", " </a>\n", "</center>\n", "\n", "<div style=\"text-align: right;\">\n", " (from <a href=\"https://www.aclweb.org/anthology/K17-1034\">Levy et al., 2017</a>; <a href=\"https://levyomer.files.wordpress.com/2017/08/zeroshot-relation-extraction-via-reading-comprehension-conll-2017.pptx\">slides</a>)\n", "</div>" ] }, { "cell_type": "markdown", "metadata": { "slideshow": { "slide_type": "slide" } }, "source": [ "## Summary\n", "\n", "* Relation extraction can be cast as question answering (and vice versa)\n", "* Information retrieval-based question answering require reading comprehension\n", "* Knowledge-based question answering requires semantic parsing" ] }, { "cell_type": "markdown", "metadata": { "slideshow": { "slide_type": "subslide" } }, "source": [ "## Background Material\n", "\n", "* Jurafky, Dan and Martin, James H. (2016). [Speech and Language Processing, Chapter 23 (Question Answering)](https://web.stanford.edu/~jurafsky/slp3/23.pdf)" ] }, { "cell_type": "markdown", "metadata": { "slideshow": { "slide_type": "slide" } }, "source": [ "## Further Reading\n", "\n", "* [Question Answering. Blog post by Vered Shwartz](http://veredshwartz.blogspot.com/2016/11/question-answering.html)\n", "* Rogers et al., 2022. [QA Dataset Explosion: A Taxonomy of NLP Resources for Question Answering and Reading Comprehension](https://dl.acm.org/doi/10.1145/3560260)\n", "* Levy et al., 2017. [Zero-Shot Relation Extraction via Reading Comprehension](https://www.aclweb.org/anthology/K17-1034)\n", "* Abdou et al., 2019. [X-WikiRE: A large, multilingual resource for relation extraction as machine comprehension](https://aclanthology.org/D19-6130/)\n", "* [AllenNLP Guide: Semantic Parsing: Intro and Seq2Seq Model](https://guide.allennlp.org/semantic-parsing-seq2seq)" ] } ], "metadata": { "celltoolbar": "Slideshow", "kernelspec": { "display_name": "Python 3", "language": "python", "name": "python3" }, "language_info": { "codemirror_mode": { "name": "ipython", "version": 3 }, "file_extension": ".py", "mimetype": "text/x-python", "name": "python", "nbconvert_exporter": "python", "pygments_lexer": "ipython3", "version": "3.8.2" } }, "nbformat": 4, "nbformat_minor": 1 }