<!DOCTYPE html> <html> <head> <meta name="databricks-html-version" content="1"> <title>055_KeystrokeBiometric - Databricks</title> <meta charset="utf-8"> <meta name="google" content="notranslate"> <meta http-equiv="Content-Language" content="en"> <meta http-equiv="Content-Type" content="text/html; charset=UTF8"> <link rel="stylesheet" href="https://fonts.googleapis.com/css?family=Source+Code+Pro:400,700"> <link rel="stylesheet" type="text/css" href="https://databricks-prod-cloudfront.cloud.databricks.com/static/201602081754420800-0c2673ac858e227cad536fdb45d140aeded238db/lib/css/bootstrap.min.css"> <link rel="stylesheet" type="text/css" href="https://databricks-prod-cloudfront.cloud.databricks.com/static/201602081754420800-0c2673ac858e227cad536fdb45d140aeded238db/lib/jquery-ui-bundle/jquery-ui.min.css"> <link rel="stylesheet" type="text/css" href="https://databricks-prod-cloudfront.cloud.databricks.com/static/201602081754420800-0c2673ac858e227cad536fdb45d140aeded238db/css/main.css"> <link rel="stylesheet" href="https://databricks-prod-cloudfront.cloud.databricks.com/static/201602081754420800-0c2673ac858e227cad536fdb45d140aeded238db/css/print.css" media="print"> <link rel="icon" type="image/png" href="https://databricks-prod-cloudfront.cloud.databricks.com/static/201602081754420800-0c2673ac858e227cad536fdb45d140aeded238db/img/favicon.ico"/> <script>window.settings = {"sparkDocsSearchGoogleCx":"004588677886978090460:_rj0wilqwdm","dbcForumURL":"http://forums.databricks.com/","dbfsS3Host":"https://databricks-prod-storage-sydney.s3.amazonaws.com","enableThirdPartyApplicationsUI":false,"enableClusterAcls":false,"notebookRevisionVisibilityHorizon":0,"enableTableHandler":true,"isAdmin":true,"enableLargeResultDownload":false,"nameAndEmail":"Raazesh Sainudiin (r.sainudiin@math.canterbury.ac.nz)","enablePresentationTimerConfig":true,"enableFullTextSearch":true,"enableElasticSparkUI":true,"clusters":true,"hideOffHeapCache":false,"applications":false,"useStaticGuide":false,"fileStoreBase":"FileStore","configurableSparkOptionsSpec":[{"keyPattern":"spark\\.kryo(\\.[^\\.]+)+","valuePattern":".*","keyPatternDisplay":"spark.kryo.*","valuePatternDisplay":"*","description":"Configuration options for Kryo serialization"},{"keyPattern":"spark\\.io\\.compression\\.codec","valuePattern":"(lzf|snappy|org\\.apache\\.spark\\.io\\.LZFCompressionCodec|org\\.apache\\.spark\\.io\\.SnappyCompressionCodec)","keyPatternDisplay":"spark.io.compression.codec","valuePatternDisplay":"snappy|lzf","description":"The codec used to compress internal data such as RDD partitions, broadcast variables and shuffle outputs."},{"keyPattern":"spark\\.serializer","valuePattern":"(org\\.apache\\.spark\\.serializer\\.JavaSerializer|org\\.apache\\.spark\\.serializer\\.KryoSerializer)","keyPatternDisplay":"spark.serializer","valuePatternDisplay":"org.apache.spark.serializer.JavaSerializer|org.apache.spark.serializer.KryoSerializer","description":"Class to use for serializing objects that will be sent over the network or need to be cached in serialized form."},{"keyPattern":"spark\\.rdd\\.compress","valuePattern":"(true|false)","keyPatternDisplay":"spark.rdd.compress","valuePatternDisplay":"true|false","description":"Whether to compress serialized RDD partitions (e.g. for StorageLevel.MEMORY_ONLY_SER). Can save substantial space at the cost of some extra CPU time."},{"keyPattern":"spark\\.speculation","valuePattern":"(true|false)","keyPatternDisplay":"spark.speculation","valuePatternDisplay":"true|false","description":"Whether to use speculation (recommended off for streaming)"},{"keyPattern":"spark\\.es(\\.[^\\.]+)+","valuePattern":".*","keyPatternDisplay":"spark.es.*","valuePatternDisplay":"*","description":"Configuration options for ElasticSearch"},{"keyPattern":"es(\\.([^\\.]+))+","valuePattern":".*","keyPatternDisplay":"es.*","valuePatternDisplay":"*","description":"Configuration options for ElasticSearch"},{"keyPattern":"spark\\.(storage|shuffle)\\.memoryFraction","valuePattern":"0?\\.0*([1-9])([0-9])*","keyPatternDisplay":"spark.(storage|shuffle).memoryFraction","valuePatternDisplay":"(0.0,1.0)","description":"Fraction of Java heap to use for Spark's shuffle or storage"},{"keyPattern":"spark\\.streaming\\.backpressure\\.enabled","valuePattern":"(true|false)","keyPatternDisplay":"spark.streaming.backpressure.enabled","valuePatternDisplay":"true|false","description":"Enables or disables Spark Streaming's internal backpressure mechanism (since 1.5). This enables the Spark Streaming to control the receiving rate based on the current batch scheduling delays and processing times so that the system receives only as fast as the system can process. Internally, this dynamically sets the maximum receiving rate of receivers. This rate is upper bounded by the values `spark.streaming.receiver.maxRate` and `spark.streaming.kafka.maxRatePerPartition` if they are set."},{"keyPattern":"spark\\.streaming\\.receiver\\.maxRate","valuePattern":"^([0-9]{1,})$","keyPatternDisplay":"spark.streaming.receiver.maxRate","valuePatternDisplay":"numeric","description":"Maximum rate (number of records per second) at which each receiver will receive data. Effectively, each stream will consume at most this number of records per second. Setting this configuration to 0 or a negative number will put no limit on the rate. See the deployment guide in the Spark Streaming programing guide for mode details."},{"keyPattern":"spark\\.streaming\\.kafka\\.maxRatePerPartition","valuePattern":"^([0-9]{1,})$","keyPatternDisplay":"spark.streaming.kafka.maxRatePerPartition","valuePatternDisplay":"numeric","description":"Maximum rate (number of records per second) at which data will be read from each Kafka partition when using the Kafka direct stream API introduced in Spark 1.3. See the Kafka Integration guide for more details."},{"keyPattern":"spark\\.streaming\\.kafka\\.maxRetries","valuePattern":"^([0-9]{1,})$","keyPatternDisplay":"spark.streaming.kafka.maxRetries","valuePatternDisplay":"numeric","description":"Maximum number of consecutive retries the driver will make in order to find the latest offsets on the leader of each partition (a default value of 1 means that the driver will make a maximum of 2 attempts). Only applies to the Kafka direct stream API introduced in Spark 1.3."},{"keyPattern":"spark\\.streaming\\.ui\\.retainedBatches","valuePattern":"^([0-9]{1,})$","keyPatternDisplay":"spark.streaming.ui.retainedBatches","valuePatternDisplay":"numeric","description":"How many batches the Spark Streaming UI and status APIs remember before garbage collecting."}],"enableReactNotebookComments":true,"enableResetPassword":true,"enableJobsSparkUpgrade":true,"sparkVersions":[{"key":"1.3.x-ubuntu15.10","displayName":"Spark 1.3.0","packageLabel":"spark-1.3-jenkins-ip-10-30-9-162-U0c2673ac85-Sa2ee4664b2-2016-02-09-02:05:59.455061","upgradable":true,"deprecated":false,"customerVisible":true},{"key":"1.4.x-ubuntu15.10","displayName":"Spark 1.4.1","packageLabel":"spark-1.4-jenkins-ip-10-30-9-162-U0c2673ac85-S33a1e4b9c6-2016-02-09-02:05:59.455061","upgradable":true,"deprecated":false,"customerVisible":true},{"key":"1.5.x-ubuntu15.10","displayName":"Spark 1.5.2","packageLabel":"spark-1.5-jenkins-ip-10-30-9-162-U0c2673ac85-S5917a1044d-2016-02-09-02:05:59.455061","upgradable":true,"deprecated":false,"customerVisible":true},{"key":"1.6.x-ubuntu15.10","displayName":"Spark 1.6.0","packageLabel":"spark-1.6-jenkins-ip-10-30-9-162-U0c2673ac85-Scabba801f3-2016-02-09-02:05:59.455061","upgradable":true,"deprecated":false,"customerVisible":true},{"key":"master","displayName":"Spark master (dev)","packageLabel":"","upgradable":true,"deprecated":false,"customerVisible":false}],"enableRestrictedClusterCreation":false,"enableFeedback":false,"defaultNumWorkers":8,"serverContinuationTimeoutMillis":10000,"driverStderrFilePrefix":"stderr","driverStdoutFilePrefix":"stdout","enableSparkDocsSearch":true,"prefetchSidebarNodes":true,"sparkHistoryServerEnabled":true,"sanitizeMarkdownHtml":true,"enableIPythonImportExport":true,"enableNotebookHistoryDiffing":true,"branch":"2.12.3","accountsLimit":-1,"enableNotebookGitBranching":true,"local":false,"displayDefaultContainerMemoryGB":6,"deploymentMode":"production","useSpotForWorkers":false,"enableUserInviteWorkflow":false,"enableStaticNotebooks":true,"dbcGuideURL":"#workspace/databricks_guide/00 Welcome to Databricks","enableCssTransitions":true,"pricingURL":"https://databricks.com/product/pricing","enableClusterAclsConfig":false,"orgId":0,"enableNotebookGitVersioning":true,"files":"files/","enableDriverLogsUI":true,"disableLegacyDashboards":false,"enableWorkspaceAclsConfig":true,"dropzoneMaxFileSize":4096,"enableNewDashboardViews":false,"driverLog4jFilePrefix":"log4j","enableMavenLibraries":true,"displayRowLimit":1000,"defaultSparkVersion":{"key":"1.5.x-ubuntu15.10","displayName":"Spark 1.5.2","packageLabel":"spark-1.5-jenkins-ip-10-30-9-162-U0c2673ac85-S5917a1044d-2016-02-09-02:05:59.455061","upgradable":true,"deprecated":false,"customerVisible":true},"clusterPublisherRootId":5,"enableLatestJobRunResultPermalink":true,"disallowAddingAdmins":false,"enableSparkConfUI":true,"enableOrgSwitcherUI":false,"clustersLimit":-1,"enableJdbcImport":true,"logfiles":"logfiles/","enableWebappSharding":false,"enableClusterDeltaUpdates":true,"csrfToken":"4c37e4ff-a908-4b05-8c61-2b76819fa34c","useFixedStaticNotebookVersionForDevelopment":false,"enableBasicReactDialogBoxes":true,"requireEmailUserName":true,"enableDashboardViews":false,"dbcFeedbackURL":"http://feedback.databricks.com/forums/263785-product-feedback","enableWorkspaceAclService":true,"someName":"Raazesh Sainudiin","enableWorkspaceAcls":true,"gitHash":"0c2673ac858e227cad536fdb45d140aeded238db","userFullname":"Raazesh Sainudiin","enableClusterCreatePage":false,"enableImportFromUrl":true,"enableMiniClusters":false,"enableWebSocketDeltaUpdates":true,"enableDebugUI":false,"showHiddenSparkVersions":false,"allowNonAdminUsers":true,"userId":100005,"dbcSupportURL":"","staticNotebookResourceUrl":"https://databricks-prod-cloudfront.cloud.databricks.com/static/201602081754420800-0c2673ac858e227cad536fdb45d140aeded238db/","enableSparkPackages":true,"enableHybridClusterType":false,"enableNotebookHistoryUI":true,"availableWorkspaces":[{"name":"Workspace 0","orgId":0}],"enableFolderHtmlExport":true,"enableSparkVersionsUI":true,"databricksGuideStaticUrl":"","enableHybridClusters":true,"notebookLoadingBackground":"#fff","enableNewJobRunDetailsPage":true,"enableDashboardExport":true,"user":"r.sainudiin@math.canterbury.ac.nz","enableServerAutoComplete":true,"enableStaticHtmlImport":true,"defaultMemoryPerContainerMB":6000,"enablePresenceUI":true,"tablesPublisherRootId":7,"enableNewInputWidgetUI":false,"accounts":true,"enableNewProgressReportUI":true,"defaultCoresPerContainer":4};</script> <script>var __DATABRICKS_NOTEBOOK_MODEL = {"version":"NotebookV1","origId":123622,"name":"055_KeystrokeBiometric","language":"scala","commands":[{"version":"CommandV1","origId":123692,"guid":"c7a300c4-36c5-4963-97be-bfea1013f77a","subtype":"command","commandType":"auto","position":0.5,"command":"%md\n\n# [Scalable Data Science](http://www.math.canterbury.ac.nz/~r.sainudiin/courses/ScalableDataScience/)\n\n## Keystroke Biometric\n### Scalable data science project by [Andrey Konstantinov](https://www.linkedin.com/in/andrey-konstantinov-38234531)\n\n*supported by* [](https://databricks.com/)\nand \n[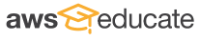](https://www.awseducate.com/microsite/CommunitiesEngageHome)","commandVersion":0,"state":"finished","results":null,"errorSummary":null,"error":null,"startTime":0.0,"submitTime":1.465877546818E12,"finishTime":0.0,"collapsed":false,"bindings":{},"inputWidgets":{},"displayType":"table","width":"auto","height":"auto","xColumns":null,"yColumns":null,"pivotColumns":null,"pivotAggregation":null,"customPlotOptions":{},"commentThread":[],"commentsVisible":false,"parentHierarchy":[],"diffInserts":[],"diffDeletes":[],"globalVars":{},"latestUser":"ako60@uclive.ac.nz","commandTitle":"","showCommandTitle":false,"hideCommandCode":false,"hideCommandResult":false,"iPythonMetadata":null,"nuid":"8f70e57d-8a11-4380-a852-04c4c0a469ca"},{"version":"CommandV1","origId":140762,"guid":"63010bc5-11bc-4b24-a158-90ae9d7c70e6","subtype":"command","commandType":"auto","position":0.75,"command":"%md\nThe [html source url](https://raw.githubusercontent.com/raazesh-sainudiin/scalable-data-science/master/db/studentProjects/08_AndreyKonstantinov/055_KeystrokeBiometric.html) of this databricks notebook and its recorded Uji :\n\n[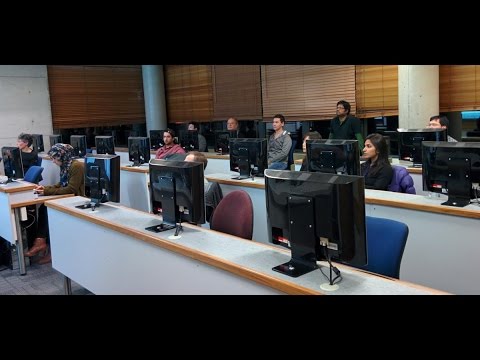](https://www.youtube.com/v/rnpa6YsDXWY?rel=0&autoplay=1&modestbranding=1&start=2586&end=3956)\n","commandVersion":0,"state":"error","results":null,"errorSummary":null,"error":null,"startTime":0.0,"submitTime":0.0,"finishTime":0.0,"collapsed":false,"bindings":{},"inputWidgets":{},"displayType":"table","width":"auto","height":"auto","xColumns":null,"yColumns":null,"pivotColumns":null,"pivotAggregation":null,"customPlotOptions":{},"commentThread":[],"commentsVisible":false,"parentHierarchy":[],"diffInserts":[],"diffDeletes":[],"globalVars":{},"latestUser":"","commandTitle":"","showCommandTitle":false,"hideCommandCode":false,"hideCommandResult":false,"iPythonMetadata":null,"nuid":"488e6a64-2b25-4d18-b066-07d3cb07125a"},{"version":"CommandV1","origId":123624,"guid":"d3ba0ef0-28e2-4f1d-9669-aae5a045fb20","subtype":"command","commandType":"auto","position":1.0,"command":"%md\n# Keystroke Biometric\nCompleted by Andrey Konstantinov for Scalable Data Science course for University of Canterbury, Christchurch\n2016/05\n\n### What is keystroke biometric?\nhttps://en.wikipedia.org/wiki/Keystroke_dynamics\n\n### Why?\n- detect if an account is shared by multiple people (to enforce licensing)\n- detect if an account is accidently used by a second person when a device is left unattended (to block it / reask for password)\n- waive the second form authentication factor for less-risky opertions (when keystroke dynamic is strong)\n- categorise PC user to experienced and not experienced\n\n### Existing work\nComparing Anomaly-Detection Algorithms for Keystroke Dynamics\n- http://www.cs.cmu.edu/~maxion/pubs/KillourhyMaxion09.pdf\n- http://www.cs.cmu.edu/~keystroke/\n\n### Related (mouse movements dynamics)\n- bots filtering (https://www.google.com/recaptcha/intro/index.html)\n- sliding bar on signin form (http://www.aliexpress.com/)\n\n### Scope of this notebook\n- overview of the data source\n- statistical evaluation of an algorithm (improved anomaly detection algorithm based on Manhattan distance from average)\n- simulation of 'creditability score' to reduce error rate\n- results review\n\n### Source code (scala) and interactive demo application (typescript/javascript)\n- https://gitlab.com/avkonst/keystroke-dynamics-course\n","commandVersion":0,"state":"finished","results":null,"errorSummary":null,"error":null,"startTime":0.0,"submitTime":1.465877546867E12,"finishTime":0.0,"collapsed":false,"bindings":{},"inputWidgets":{},"displayType":"table","width":"auto","height":"auto","xColumns":null,"yColumns":null,"pivotColumns":null,"pivotAggregation":null,"customPlotOptions":{},"commentThread":[],"commentsVisible":false,"parentHierarchy":[],"diffInserts":[],"diffDeletes":[],"globalVars":{},"latestUser":"ako60@uclive.ac.nz","commandTitle":"","showCommandTitle":false,"hideCommandCode":false,"hideCommandResult":false,"iPythonMetadata":null,"nuid":"ba201580-dcdd-480b-bc9d-653c7fdf4c72"},{"version":"CommandV1","origId":124830,"guid":"4a44a729-0a83-44ee-8162-e04a0497daca","subtype":"command","commandType":"auto","position":2.0,"command":"// NEEDED TO MOVE EVERYTHING TO ONE CELL TO WORKAROUND DATABRICKS COMPILATION ERROR\n// so all documentation is in embeded comments\n\n// load data source file (50 people, typed the same password 400 times, in 8 sessions)\nimport scala.io.Source\nval datasource = Source.fromURL(\"https://gitlab.com/avkonst/keystroke-dynamics-course/raw/master/data/DSL-StrongPasswordData.csv\").getLines()\n\n// supportive case classes to handle input rows and results\ncase class Row(subject: String, session: Int, attempt: Int, timings: Array[Double])\ncase class Result(subject: String, threshold: Double,\n falseNegativesErrorRate: Double,\n falsePositivesErrorRate: Double,\n falseNegativesErrorSimulation: Double,\n falsePositivesErrorSimulation: Double,\n consistencyLevel: Double\n )\n\nval rows = datasource\n.drop(1) // skip header\n.map(i => i.split(\",\")) // split into columns\n.map(i => Row(i(0), i(1).toInt, i(2).toInt, i.drop(3).map(j => j.toDouble))) // extract rows data\n.toArray\n\n// supportive functions\n\n // returns factors to normalize timings data to the range from 0 to 1\n def getTimingsFactors(data: Array[Row]): Array[Double] = {\n val timingFactors = (0 until data.head.timings.length).map(i => 1.0).toArray\n (0 until data.head.timings.length).foreach(index => {\n data.foreach(row => {\n timingFactors(index) = Math.max(row.timings(index), timingFactors(index))\n })\n })\n timingFactors.map(i => 1.0/i)\n }\n\n // returns a distance between 2 vectors (using Manhattan distance and normalization factors)\n def getDistance(array1: Array[Double], array2: Array[Double], timingFactors: Array[Double]) = {\n var maxDistance = 0D\n val sum = (0 until array1.length).map(colInd => {\n val currentColDistance = Math.abs(array1(colInd) - array2(colInd)) * timingFactors(colInd)\n maxDistance = Math.max(maxDistance, currentColDistance)\n currentColDistance\n }).toArray.sum\n sum - maxDistance * 2 // maxDistance * 2 is for noise reduction, selected emprically\n }\n\n // returns an average vector of timings for the set of provided rows\n def getAverageTimings(data: Array[Row]) = {\n val sumTimings = data.head.timings.map(i => 0D)\n data.foreach(row => {\n (0 until sumTimings.length).foreach(colInd => {\n sumTimings(colInd) += row.timings(colInd)\n })\n })\n sumTimings.map(i => i / data.length)\n }\n\n// higher-level supportive functions\n\n // identifies and removes outliers in rows incrementally up to the specified ratio\n def getRepresentativeSet(data: Array[Row], allowedFalseNegativeRate: Double): Array[Row] = {\n var result = data\n val numberOfRowsToRemove = (data.length * allowedFalseNegativeRate).toInt\n (0 until numberOfRowsToRemove).foreach(iteration => {\n val timingFactors = getTimingsFactors(result)\n val averageTimings = getAverageTimings(result)\n result = result.map(\n row => (getDistance(averageTimings, row.timings, timingFactors) -> row))\n .sortBy(i => i._1)\n .reverse\n .drop(1)\n .map(i => i._2)\n })\n result\n }\n\n // simulates a login process using 'creditability score',\n // allows to identify a case when a subject produces a 'strong' keystroke\n // and when it fails to sustain even 'weak' keystroke match continously\n def simulateCreditabilityScore(data: Array[Row], averageTimings: Array[Double], timingFactors: Array[Double], threshold: Double) = {\n var trials = 0\n var creditability = 0.0\n var blocked = false\n var interrupted = false\n data.foreach(row => {\n if (blocked == false && interrupted == false) {\n val distance = getDistance(averageTimings, row.timings, timingFactors)\n trials += 1\n if (trials > 4) {\n // when a password typed 5 times with 'weak' keystroke, return 'rejected' status\n blocked = true\n }\n else {\n if (distance > threshold) {\n creditability -= (distance / threshold - 1)\n if (creditability < -1.0) {\n // when creditability drops significantly, return 'rejected' status\n blocked = true\n }\n }\n else {\n creditability += (1 - distance / threshold)\n if (creditability > 0.15) {\n // when a password is typed with 'strong' keystroke or creditability is recovered, return 'accepted' status\n interrupted = true\n }\n }\n }\n }\n })\n blocked\n }\n\n// main routine which analyses a given subject\n\n def analyseSubject(data: Array[Row], subject: String) = {\n // get 80% of data as train data for the specified subject\n val currentSubjectTrainData = data.filter(i => i.subject == subject && i.attempt <= 40)\n // get 20% as test data for the specified subject\n val currentSubjectTestData = data.filter(i => i.subject == subject && i.attempt > 40)\n\n //\n // BUILD MODEL\n // get representative set from the train data\n val representativeSet = getRepresentativeSet(currentSubjectTrainData, 0.2)\n // calculate subject specific parameters, representing digital keystroke signature\n val timingFactors = getTimingsFactors(representativeSet)\n val averageTimings = getAverageTimings(representativeSet)\n // threshold is set to a maximum value to reach 0% false-negative error rate for the rows from representative set\n val threshold = representativeSet.map(row => getDistance(averageTimings, row.timings, timingFactors)).max\n // consistency of a subject can be estimated by the following parameter (normalized)\n val consistencyLevel = threshold / averageTimings.zipWithIndex.map(i => i._1 * timingFactors(i._2)).sum\n\n //\n // APPLY THE MODEL ON TEST DATA\n // calculate ratio of rows REJECTED by the model\n val falseNegatives = currentSubjectTestData.map(\n row => getDistance(averageTimings, row.timings, timingFactors))\n .filter(i => i > threshold)\n val falseNegativesErrorRate = falseNegatives.length.toDouble / currentSubjectTestData.length\n\n //\n // APPLY THE MODEL ON OTHER SUBJECTS\n // calculate ratio of rows ACCEPTED by the model\n val otherSubjectsData = data.filter(i => i.subject != subject /*&& i.attempt < 2 && i.session == 1*/)\n val falsePositives = otherSubjectsData.map(\n row => getDistance(averageTimings, row.timings, timingFactors))\n .filter(i => i <= threshold)\n val falsePositivesErrorRate = falsePositives.length.toDouble / otherSubjectsData.length\n\n //\n // APPLY THE MODEL FOR CREDITABILITY SCORE SIMULATION ON TEST DATA\n // calculate ratio of sessions REJECTED by the model\n val currentSubjectTestDataGrouped = currentSubjectTestData.groupBy(i => i.subject + \"_\" + i.session)\n .map(i => (i._1 -> i._2.sortBy(j => j.attempt)))\n val currentSubjectTestDataSimulatedGroups = currentSubjectTestDataGrouped.map(\n group => simulateCreditabilityScore(group._2, averageTimings, timingFactors, threshold))\n .toArray\n val falseNegativesErrorRateWithCreditability = currentSubjectTestDataSimulatedGroups.count(i => i == true).toDouble / currentSubjectTestDataSimulatedGroups.length\n\n //\n // APPLY THE MODEL FOR CREDITABILITY SCORE SIMULATION ON OTHER SUBJECTS\n // calculate ratio of sessions ACCEPTED by the model\n val otherSubjectsDataGrouped = otherSubjectsData./*filter(i => i.session == 1).*/groupBy(i => i.subject + \"_\" + i.session)\n .map(i => (i._1 -> i._2.sortBy(j => j.attempt)))\n val otherSubjectsDataSimulatedGroups = otherSubjectsDataGrouped.map(\n group => simulateCreditabilityScore(group._2, averageTimings, timingFactors, threshold))\n .toArray\n val falsePositivesErrorRateWithCreditability = otherSubjectsDataSimulatedGroups.count(i => i == false).toDouble / otherSubjectsDataSimulatedGroups.length\n\n Result(\n subject,\n threshold,\n falseNegativesErrorRate,\n falsePositivesErrorRate,\n falseNegativesErrorRateWithCreditability,\n falsePositivesErrorRateWithCreditability,\n consistencyLevel\n )\n }\n\n// get all unique subjects\nval subjects = rows.map(row => row.subject).distinct\n\n// calculate error rates per every subject\nval errorRates = subjects.map(subject => analyseSubject(rows, subject))\n .sortBy(i => i.consistencyLevel).reverse // order by consistency descending\n\n// print results per every subject\nerrorRates.foreach(i => {\n println(s\"${i.subject}: Applied threshold ${i.threshold}\")\n println(s\"${i.subject}: False Negatives Error Rate ${i.falseNegativesErrorRate}\")\n println(s\"${i.subject}: False Positives Error Rate ${i.falsePositivesErrorRate}\")\n println(s\"${i.subject}: False Negatives Scores Simulation ${i.falseNegativesErrorSimulation}\")\n println(s\"${i.subject}: False Positives Scores Simulation ${i.falsePositivesErrorSimulation}\")\n println(s\"${i.subject}: Consistency level ${i.consistencyLevel}\")\n println(\"\")\n })\n\n// print cummulative result\n// note: calculating average over error rates and anomaly scores does not make much sense, but it is good enough to compare error rates of 2 different methods\nval errorRatesForConsistent = errorRates//.filter(i => i.consistencyLevel < 0.27)\nprintln(s\"TOTAL: False Negatives ${errorRatesForConsistent.map(i => i.falseNegativesErrorRate).sum / errorRatesForConsistent.length}\")\nprintln(s\"TOTAL: False Positives ${errorRatesForConsistent.map(i => i.falsePositivesErrorRate).sum / errorRatesForConsistent.length}\")\nprintln(s\"TOTAL: False Negatives Simulation ${errorRatesForConsistent.map(i => i.falseNegativesErrorSimulation).sum / errorRatesForConsistent.length}\")\nprintln(s\"TOTAL: False Positives Simulation ${errorRatesForConsistent.map(i => i.falsePositivesErrorSimulation).sum / errorRatesForConsistent.length}\")","commandVersion":0,"state":"finished","results":{"type":"html","data":"<div class=\"ansiout\">s020: Applied threshold 1.2773733159529799\ns020: False Negatives Error Rate 0.15\ns020: False Positives Error Rate 0.598\ns020: False Negatives Scores Simulation 0.125\ns020: False Positives Scores Simulation 0.4525\ns020: Consistency level 0.3852353878543283\n\ns049: Applied threshold 1.5610245797249591\ns049: False Negatives Error Rate 0.175\ns049: False Positives Error Rate 0.34025\ns049: False Negatives Scores Simulation 0.125\ns049: False Positives Scores Simulation 0.34\ns049: Consistency level 0.3793239190307475\n\ns040: Applied threshold 1.6911959491290878\ns040: False Negatives Error Rate 0.2\ns040: False Positives Error Rate 0.2587\ns040: False Negatives Scores Simulation 0.125\ns040: False Positives Scores Simulation 0.2225\ns040: Consistency level 0.316363916748968\n\ns003: Applied threshold 1.0476080398348857\ns003: False Negatives Error Rate 0.1875\ns003: False Positives Error Rate 0.22\ns003: False Negatives Scores Simulation 0.0\ns003: False Positives Scores Simulation 0.075\ns003: Consistency level 0.2986876829253832\n\ns011: Applied threshold 0.9377496093750001\ns011: False Negatives Error Rate 0.1875\ns011: False Positives Error Rate 0.095\ns011: False Negatives Scores Simulation 0.125\ns011: False Positives Scores Simulation 0.03\ns011: Consistency level 0.2979422510768676\n\ns016: Applied threshold 1.6007095971490837\ns016: False Negatives Error Rate 0.1\ns016: False Positives Error Rate 0.06355\ns016: False Negatives Scores Simulation 0.125\ns016: False Positives Scores Simulation 0.055\ns016: Consistency level 0.29315699116875016\n\ns047: Applied threshold 1.3243246111732865\ns047: False Negatives Error Rate 0.225\ns047: False Positives Error Rate 0.23255\ns047: False Negatives Scores Simulation 0.25\ns047: False Positives Scores Simulation 0.18\ns047: Consistency level 0.2854641616364273\n\ns035: Applied threshold 1.102367579807221\ns035: False Negatives Error Rate 0.0875\ns035: False Positives Error Rate 0.31445\ns035: False Negatives Scores Simulation 0.125\ns035: False Positives Scores Simulation 0.2175\ns035: Consistency level 0.2828022648152238\n\ns033: Applied threshold 1.5676547712364537\ns033: False Negatives Error Rate 0.1625\ns033: False Positives Error Rate 0.06355\ns033: False Negatives Scores Simulation 0.125\ns033: False Positives Scores Simulation 0.0675\ns033: Consistency level 0.27654056841782493\n\ns057: Applied threshold 0.8799113281250006\ns057: False Negatives Error Rate 0.15\ns057: False Positives Error Rate 0.23825\ns057: False Negatives Scores Simulation 0.0\ns057: False Positives Scores Simulation 0.16\ns057: Consistency level 0.272443507449229\n\ns041: Applied threshold 1.0125387388192428\ns041: False Negatives Error Rate 0.125\ns041: False Positives Error Rate 0.0624\ns041: False Negatives Scores Simulation 0.375\ns041: False Positives Scores Simulation 0.0225\ns041: Consistency level 0.2689758222952891\n\ns032: Applied threshold 0.8967103073605761\ns032: False Negatives Error Rate 0.2875\ns032: False Positives Error Rate 0.3911\ns032: False Negatives Scores Simulation 0.125\ns032: False Positives Scores Simulation 0.2725\ns032: Consistency level 0.26868391954648063\n\ns031: Applied threshold 1.1132214018404674\ns031: False Negatives Error Rate 0.2375\ns031: False Positives Error Rate 0.2959\ns031: False Negatives Scores Simulation 0.125\ns031: False Positives Scores Simulation 0.215\ns031: Consistency level 0.26591019817145756\n\ns051: Applied threshold 0.8502374999999995\ns051: False Negatives Error Rate 0.2125\ns051: False Positives Error Rate 0.2117\ns051: False Negatives Scores Simulation 0.125\ns051: False Positives Scores Simulation 0.1475\ns051: Consistency level 0.26206643792024564\n\ns034: Applied threshold 0.8844475056534284\ns034: False Negatives Error Rate 0.25\ns034: False Positives Error Rate 0.21155\ns034: False Negatives Scores Simulation 0.125\ns034: False Positives Scores Simulation 0.165\ns034: Consistency level 0.26021019814382396\n\ns015: Applied threshold 0.8093712335643646\ns015: False Negatives Error Rate 0.3\ns015: False Positives Error Rate 0.1392\ns015: False Negatives Scores Simulation 0.125\ns015: False Positives Scores Simulation 0.14\ns015: Consistency level 0.256853144261177\n\ns002: Applied threshold 0.963913928310338\ns002: False Negatives Error Rate 0.175\ns002: False Positives Error Rate 0.2981\ns002: False Negatives Scores Simulation 0.25\ns002: False Positives Scores Simulation 0.175\ns002: Consistency level 0.2533316602779885\n\ns018: Applied threshold 0.9793204306081632\ns018: False Negatives Error Rate 0.1625\ns018: False Positives Error Rate 0.18535\ns018: False Negatives Scores Simulation 0.125\ns018: False Positives Scores Simulation 0.0925\ns018: Consistency level 0.2512053325367009\n\ns022: Applied threshold 1.3952974947277794\ns022: False Negatives Error Rate 0.1625\ns022: False Positives Error Rate 0.0169\ns022: False Negatives Scores Simulation 0.0\ns022: False Positives Scores Simulation 0.0025\ns022: Consistency level 0.250629044702529\n\ns036: Applied threshold 1.4926723057428783\ns036: False Negatives Error Rate 0.15\ns036: False Positives Error Rate 0.0012\ns036: False Negatives Scores Simulation 0.125\ns036: False Positives Scores Simulation 0.0\ns036: Consistency level 0.24866315657168128\n\ns007: Applied threshold 0.755808657541738\ns007: False Negatives Error Rate 0.2\ns007: False Positives Error Rate 0.17755\ns007: False Negatives Scores Simulation 0.25\ns007: False Positives Scores Simulation 0.1225\ns007: Consistency level 0.24529621582479325\n\ns012: Applied threshold 0.960161167580198\ns012: False Negatives Error Rate 0.1375\ns012: False Positives Error Rate 0.06505\ns012: False Negatives Scores Simulation 0.0\ns012: False Positives Scores Simulation 0.0225\ns012: Consistency level 0.24133987216822197\n\ns056: Applied threshold 0.8017226562499999\ns056: False Negatives Error Rate 0.2375\ns056: False Positives Error Rate 0.12645\ns056: False Negatives Scores Simulation 0.0\ns056: False Positives Scores Simulation 0.0625\ns056: Consistency level 0.2394794206689195\n\ns052: Applied threshold 1.137289883439248\ns052: False Negatives Error Rate 0.2125\ns052: False Positives Error Rate 0.0028\ns052: False Negatives Scores Simulation 0.125\ns052: False Positives Scores Simulation 0.0\ns052: Consistency level 0.23897403575537088\n\ns046: Applied threshold 1.0582540745489628\ns046: False Negatives Error Rate 0.2\ns046: False Positives Error Rate 0.1334\ns046: False Negatives Scores Simulation 0.125\ns046: False Positives Scores Simulation 0.08\ns046: Consistency level 0.2365657561461832\n\ns008: Applied threshold 0.7613779613559349\ns008: False Negatives Error Rate 0.1875\ns008: False Positives Error Rate 0.12785\ns008: False Negatives Scores Simulation 0.125\ns008: False Positives Scores Simulation 0.1075\ns008: Consistency level 0.23029609471251\n\ns043: Applied threshold 1.177606191023883\ns043: False Negatives Error Rate 0.1125\ns043: False Positives Error Rate 0.0142\ns043: False Negatives Scores Simulation 0.125\ns043: False Positives Scores Simulation 0.005\ns043: Consistency level 0.229758574811945\n\ns027: Applied threshold 1.0010160577417553\ns027: False Negatives Error Rate 0.1\ns027: False Positives Error Rate 0.0963\ns027: False Negatives Scores Simulation 0.125\ns027: False Positives Scores Simulation 0.045\ns027: Consistency level 0.2271888194045635\n\ns037: Applied threshold 0.7253005709021437\ns037: False Negatives Error Rate 0.2625\ns037: False Positives Error Rate 0.16415\ns037: False Negatives Scores Simulation 0.125\ns037: False Positives Scores Simulation 0.0825\ns037: Consistency level 0.2239235268864702\n\ns004: Applied threshold 0.8135658544446964\ns004: False Negatives Error Rate 0.1875\ns004: False Positives Error Rate 0.11995\ns004: False Negatives Scores Simulation 0.0\ns004: False Positives Scores Simulation 0.065\ns004: Consistency level 0.219099270263811\n\ns053: Applied threshold 0.7178906249999999\ns053: False Negatives Error Rate 0.15\ns053: False Positives Error Rate 0.0288\ns053: False Negatives Scores Simulation 0.125\ns053: False Positives Scores Simulation 0.0175\ns053: Consistency level 0.21528099100945902\n\ns030: Applied threshold 1.1121031525739422\ns030: False Negatives Error Rate 0.175\ns030: False Positives Error Rate 0.0208\ns030: False Negatives Scores Simulation 0.0\ns030: False Positives Scores Simulation 0.015\ns030: Consistency level 0.2130951480275123\n\ns050: Applied threshold 0.8208762328522515\ns050: False Negatives Error Rate 0.1875\ns050: False Positives Error Rate 0.1148\ns050: False Negatives Scores Simulation 0.125\ns050: False Positives Scores Simulation 0.0525\ns050: Consistency level 0.20264523964437478\n\ns055: Applied threshold 0.5433457031250001\ns055: False Negatives Error Rate 0.25\ns055: False Positives Error Rate 0.0024\ns055: False Negatives Scores Simulation 0.125\ns055: False Positives Scores Simulation 0.0\ns055: Consistency level 0.2003767927583647\n\ns021: Applied threshold 0.7669687824250512\ns021: False Negatives Error Rate 0.125\ns021: False Positives Error Rate 0.0602\ns021: False Negatives Scores Simulation 0.125\ns021: False Positives Scores Simulation 0.0175\ns021: Consistency level 0.19720745566079378\n\ns013: Applied threshold 0.5741658913580499\ns013: False Negatives Error Rate 0.2375\ns013: False Positives Error Rate 0.03765\ns013: False Negatives Scores Simulation 0.125\ns013: False Positives Scores Simulation 0.035\ns013: Consistency level 0.19346059746926916\n\ns038: Applied threshold 0.7296060784308096\ns038: False Negatives Error Rate 0.075\ns038: False Positives Error Rate 0.0149\ns038: False Negatives Scores Simulation 0.0\ns038: False Positives Scores Simulation 0.005\ns038: Consistency level 0.19278415330516857\n\ns029: Applied threshold 0.6458359950536199\ns029: False Negatives Error Rate 0.15\ns029: False Positives Error Rate 0.05245\ns029: False Negatives Scores Simulation 0.125\ns029: False Positives Scores Simulation 0.02\ns029: Consistency level 0.19252619318320283\n\ns044: Applied threshold 0.7764086185062573\ns044: False Negatives Error Rate 0.175\ns044: False Positives Error Rate 0.0126\ns044: False Negatives Scores Simulation 0.125\ns044: False Positives Scores Simulation 0.0025\ns044: Consistency level 0.19194991790272226\n\ns048: Applied threshold 0.671234040836241\ns048: False Negatives Error Rate 0.15\ns048: False Positives Error Rate 0.0456\ns048: False Negatives Scores Simulation 0.0\ns048: False Positives Scores Simulation 0.025\ns048: Consistency level 0.19097978977656802\n\ns026: Applied threshold 0.7262589206744209\ns026: False Negatives Error Rate 0.2\ns026: False Positives Error Rate 0.09255\ns026: False Negatives Scores Simulation 0.0\ns026: False Positives Scores Simulation 0.0425\ns026: Consistency level 0.1882074017237553\n\ns024: Applied threshold 0.8573151296497107\ns024: False Negatives Error Rate 0.1375\ns024: False Positives Error Rate 0.017\ns024: False Negatives Scores Simulation 0.125\ns024: False Positives Scores Simulation 0.005\ns024: Consistency level 0.18723405877440852\n\ns054: Applied threshold 0.6671500241631287\ns054: False Negatives Error Rate 0.1625\ns054: False Positives Error Rate 0.04825\ns054: False Negatives Scores Simulation 0.0\ns054: False Positives Scores Simulation 0.0225\ns054: Consistency level 0.18250062550883453\n\ns028: Applied threshold 0.7346201748924511\ns028: False Negatives Error Rate 0.1875\ns028: False Positives Error Rate 0.0203\ns028: False Negatives Scores Simulation 0.0\ns028: False Positives Scores Simulation 0.0075\ns028: Consistency level 0.17742233160862106\n\ns005: Applied threshold 0.9843046874999992\ns005: False Negatives Error Rate 0.075\ns005: False Positives Error Rate 0.01725\ns005: False Negatives Scores Simulation 0.0\ns005: False Positives Scores Simulation 0.005\ns005: Consistency level 0.17449663128194642\n\ns010: Applied threshold 0.5444652343749998\ns010: False Negatives Error Rate 0.2625\ns010: False Positives Error Rate 0.00495\ns010: False Negatives Scores Simulation 0.0\ns010: False Positives Scores Simulation 0.0\ns010: Consistency level 0.1647376199855452\n\ns039: Applied threshold 0.5134987251183448\ns039: False Negatives Error Rate 0.175\ns039: False Positives Error Rate 0.0136\ns039: False Negatives Scores Simulation 0.25\ns039: False Positives Scores Simulation 0.0\ns039: Consistency level 0.15926721468697938\n\ns019: Applied threshold 0.7057269855505732\ns019: False Negatives Error Rate 0.125\ns019: False Positives Error Rate 3.5E-4\ns019: False Negatives Scores Simulation 0.0\ns019: False Positives Scores Simulation 0.0\ns019: Consistency level 0.15855657596806447\n\ns017: Applied threshold 0.5358350346037614\ns017: False Negatives Error Rate 0.1875\ns017: False Positives Error Rate 0.00475\ns017: False Negatives Scores Simulation 0.125\ns017: False Positives Scores Simulation 0.005\ns017: Consistency level 0.152714215390689\n\ns025: Applied threshold 0.6800326408152348\ns025: False Negatives Error Rate 0.275\ns025: False Positives Error Rate 0.0065\ns025: False Negatives Scores Simulation 0.125\ns025: False Positives Scores Simulation 0.005\ns025: Consistency level 0.14918255906996006\n\ns042: Applied threshold 0.5834686846173982\ns042: False Negatives Error Rate 0.15\ns042: False Positives Error Rate 1.5E-4\ns042: False Negatives Scores Simulation 0.125\ns042: False Positives Scores Simulation 0.0\ns042: Consistency level 0.14209711922047263\n\nTOTAL: False Negatives 0.1791666666666667\nTOTAL: False Positives 0.11531862745098038\nTOTAL: False Negatives Simulation 0.10294117647058823\nTOTAL: False Positives Simulation 0.07661764705882351\nimport scala.io.Source\ndatasource: Iterator[String] = empty iterator\ndefined class Row\ndefined class Result\nrows: Array[Row] = Array(Row(s002,1,1,[D@1aca7ee2), Row(s002,1,2,[D@64e87fb0), Row(s002,1,3,[D@756ddab2), Row(s002,1,4,[D@399670f4), Row(s002,1,5,[D@70e2344a), Row(s002,1,6,[D@5750c019), Row(s002,1,7,[D@4c73380b), Row(s002,1,8,[D@4668e0a1), Row(s002,1,9,[D@27c9dc42), Row(s002,1,10,[D@764880aa), Row(s002,1,11,[D@23ae4d08), Row(s002,1,12,[D@2242dc34), Row(s002,1,13,[D@4b003ff2), Row(s002,1,14,[D@79ffabe8), Row(s002,1,15,[D@28e87fd2), Row(s002,1,16,[D@1c7fa587), Row(s002,1,17,[D@56caf655), Row(s002,1,18,[D@526eb78f), Row(s002,1,19,[D@391344ff), Row(s002,1,20,[D@58a18dc7), Row(s002,1,21,[D@59d8b0d8), Row(s002,1,22,[D@7e2db6a3), Row(s002,1,23,[D@5dba2125), Row(s002,1,24,[D@9a150e0), Row(s002,1,25,[D@15e36f7c), Row(s002,1,26,[D@6e84bcd), Row(s002,1,27,[D@ad7f13c), Row(s002,1,28,[D@97296d6), Row(s002,1,29,[D@764800b), Row(s002,1,30,[D@43960509), Row(s002,1,31,[D@76fd5256), Row(s002,1,32,[D@6ba4ae79), Row(s002,1,33,[D@48631fde), Row(s002,1,34,[D@3baef596), Row(s002,1,35,[D@27dd6f57), Row(s002,1,36,[D@6944cab3), Row(s002,1,37,[D@3909a5aa), Row(s002,1,38,[D@75c7e558), Row(s002,1,39,[D@392c8b60), Row(s002,1,40,[D@76f2dfa4), Row(s002,1,41,[D@2a20025), Row(s002,1,42,[D@4885c80c), Row(s002,1,43,[D@152f2df7), Row(s002,1,44,[D@75c0df09), Row(s002,1,45,[D@67714abc), Row(s002,1,46,[D@1523bf6), Row(s002,1,47,[D@6d7eeebe), Row(s002,1,48,[D@1eb54c8f), Row(s002,1,49,[D@73fcd14f), Row(s002,1,50,[D@63e1c94d), Row(s002,2,1,[D@7970d091), Row(s002,2,2,[D@4d952a04), Row(s002,2,3,[D@58f6ec50), Row(s002,2,4,[D@3530fda2), Row(s002,2,5,[D@4abb47cd), Row(s002,2,6,[D@2e1a9194), Row(s002,2,7,[D@41e17c6b), Row(s002,2,8,[D@730e5418), Row(s002,2,9,[D@639be09b), Row(s002,2,10,[D@19775b4d), Row(s002,2,11,[D@53bf6e2c), Row(s002,2,12,[D@52905ed7), Row(s002,2,13,[D@6e33e326), Row(s002,2,14,[D@4dbd609e), Row(s002,2,15,[D@65dbb90b), Row(s002,2,16,[D@35ed3581), Row(s002,2,17,[D@44b6cac9), Row(s002,2,18,[D@6229eb51), Row(s002,2,19,[D@5a8215fe), Row(s002,2,20,[D@69f7212), Row(s002,2,21,[D@748d008a), Row(s002,2,22,[D@59d31e29), Row(s002,2,23,[D@1334dd08), Row(s002,2,24,[D@6e46229e), Row(s002,2,25,[D@72d3e68c), Row(s002,2,26,[D@33308ca9), Row(s002,2,27,[D@6d9cb5a), Row(s002,2,28,[D@59544bcd), Row(s002,2,29,[D@34de31ed), Row(s002,2,30,[D@33cb61b), Row(s002,2,31,[D@4b772e3d), Row(s002,2,32,[D@30061ebd), Row(s002,2,33,[D@75120009), Row(s002,2,34,[D@1378fcde), Row(s002,2,35,[D@610c3e4a), Row(s002,2,36,[D@617f3720), Row(s002,2,37,[D@408714e), Row(s002,2,38,[D@5042f31d), Row(s002,2,39,[D@50bc6931), Row(s002,2,40,[D@48629231), Row(s002,2,41,[D@f271872), Row(s002,2,42,[D@48bac890), Row(s002,2,43,[D@7bfcc557), Row(s002,2,44,[D@27532303), Row(s002,2,45,[D@10004b13), Row(s002,2,46,[D@ee0efcb), Row(s002,2,47,[D@13ab553d), Row(s002,2,48,[D@2d5e2770), Row(s002,2,49,[D@3f94b91b), Row(s002,2,50,[D@36839795), Row(s002,3,1,[D@7fc83ec5), Row(s002,3,2,[D@23f1e3eb), Row(s002,3,3,[D@3937caef), Row(s002,3,4,[D@13a2cd63), Row(s002,3,5,[D@2d22f7c4), Row(s002,3,6,[D@1e0a730), Row(s002,3,7,[D@6077551), Row(s002,3,8,[D@34b7927), Row(s002,3,9,[D@39ed325d), Row(s002,3,10,[D@3d30cb77), Row(s002,3,11,[D@209c7d), Row(s002,3,12,[D@58fa4f1f), Row(s002,3,13,[D@8d5f187), Row(s002,3,14,[D@3305f899), Row(s002,3,15,[D@374249f0), Row(s002,3,16,[D@3dca6ab0), Row(s002,3,17,[D@1ab4ed47), Row(s002,3,18,[D@6690fb8), Row(s002,3,19,[D@23c1db4c), Row(s002,3,20,[D@4db0aa6e), Row(s002,3,21,[D@7053b84a), Row(s002,3,22,[D@3e897d37), Row(s002,3,23,[D@13bfda11), Row(s002,3,24,[D@5b14e1d2), Row(s002,3,25,[D@36e89312), Row(s002,3,26,[D@43fd303d), Row(s002,3,27,[D@2e7f0501), Row(s002,3,28,[D@52997b6d), Row(s002,3,29,[D@209b022f), Row(s002,3,30,[D@a4da2d9), Row(s002,3,31,[D@5c4ce99c), Row(s002,3,32,[D@4597a36b), Row(s002,3,33,[D@3de55b8c), Row(s002,3,34,[D@5a597d83), Row(s002,3,35,[D@61e27f5d), Row(s002,3,36,[D@199614f1), Row(s002,3,37,[D@e381574), Row(s002,3,38,[D@1f1cd455), Row(s002,3,39,[D@6df6587c), Row(s002,3,40,[D@446ee8af), Row(s002,3,41,[D@ab3c6e3), Row(s002,3,42,[D@73f4059c), Row(s002,3,43,[D@2c9e9626), Row(s002,3,44,[D@1f86388a), Row(s002,3,45,[D@b967147), Row(s002,3,46,[D@581d4d4c), Row(s002,3,47,[D@6acfcf), Row(s002,3,48,[D@2e86e520), Row(s002,3,49,[D@1622a5fe), Row(s002,3,50,[D@24e7fd5b), Row(s002,4,1,[D@72a54abf), Row(s002,4,2,[D@6b931e2e), Row(s002,4,3,[D@689d4bf7), Row(s002,4,4,[D@730b6e9a), Row(s002,4,5,[D@2b2332f6), Row(s002,4,6,[D@58b23bd2), Row(s002,4,7,[D@5af211c0), Row(s002,4,8,[D@7225dbba), Row(s002,4,9,[D@40a3f38a), Row(s002,4,10,[D@9862ce7), Row(s002,4,11,[D@43305006), Row(s002,4,12,[D@1f14abd1), Row(s002,4,13,[D@40f4241a), Row(s002,4,14,[D@78add9f7), Row(s002,4,15,[D@39dc1684), Row(s002,4,16,[D@3ac784d), Row(s002,4,17,[D@629958d6), Row(s002,4,18,[D@746d4713), Row(s002,4,19,[D@2ddc172c), Row(s002,4,20,[D@4dd27cca), Row(s002,4,21,[D@65a5d24e), Row(s002,4,22,[D@7bee4436), Row(s002,4,23,[D@36c64910), Row(s002,4,24,[D@68ac472e), Row(s002,4,25,[D@2350cfd7), Row(s002,4,26,[D@2a16f07f), Row(s002,4,27,[D@2e1ca2e4), Row(s002,4,28,[D@2403053e), Row(s002,4,29,[D@188481e), Row(s002,4,30,[D@1c00d558), Row(s002,4,31,[D@57c05fbe), Row(s002,4,32,[D@6b569a8d), Row(s002,4,33,[D@28dde741), Row(s002,4,34,[D@326d5d17), Row(s002,4,35,[D@6785c94b), Row(s002,4,36,[D@38d8a5c4), Row(s002,4,37,[D@7ff8aa71), Row(s002,4,38,[D@2725d57c), Row(s002,4,39,[D@6e239f42), Row(s002,4,40,[D@1328e1c7), Row(s002,4,41,[D@29b97af1), Row(s002,4,42,[D@20bed48f), Row(s002,4,43,[D@52158655), Row(s002,4,44,[D@6e77309), Row(s002,4,45,[D@64ebffd6), Row(s002,4,46,[D@32275568), Row(s002,4,47,[D@4cfe5a57), Row(s002,4,48,[D@713c07fa), Row(s002,4,49,[D@4a925344), Row(s002,4,50,[D@4216d36b), Row(s002,5,1,[D@7c04059c), Row(s002,5,2,[D@6d96ce31), Row(s002,5,3,[D@35c6b8b4), Row(s002,5,4,[D@413fb8ec), Row(s002,5,5,[D@1dcb7db2), Row(s002,5,6,[D@46f7cf7c), Row(s002,5,7,[D@46f4dc0e), Row(s002,5,8,[D@7ab0f4e4), Row(s002,5,9,[D@3c5020ed), Row(s002,5,10,[D@44248434), Row(s002,5,11,[D@24d02412), Row(s002,5,12,[D@59bae3b8), Row(s002,5,13,[D@6450f72a), Row(s002,5,14,[D@4b5cab0), Row(s002,5,15,[D@21e08e80), Row(s002,5,16,[D@2fc91e27), Row(s002,5,17,[D@4c435553), Row(s002,5,18,[D@6609f621), Row(s002,5,19,[D@4338f0ee), Row(s002,5,20,[D@2467e688), Row(s002,5,21,[D@72d8c69a), Row(s002,5,22,[D@5bc98e1e), Row(s002,5,23,[D@5ff2aa09), Row(s002,5,24,[D@44ba54d9), Row(s002,5,25,[D@70e2a6c2), Row(s002,5,26,[D@67cc7fbe), Row(s002,5,27,[D@2da433ac), Row(s002,5,28,[D@3b2ea65d), Row(s002,5,29,[D@5e1fc34c), Row(s002,5,30,[D@5a2a66be), Row(s002,5,31,[D@569f1704), Row(s002,5,32,[D@18cd4fc4), Row(s002,5,33,[D@38e8ea32), Row(s002,5,34,[D@317e6407), Row(s002,5,35,[D@1ff7621b), Row(s002,5,36,[D@6d36bd4e), Row(s002,5,37,[D@12f073a0), Row(s002,5,38,[D@50ec73a5), Row(s002,5,39,[D@74af2419), Row(s002,5,40,[D@2cab2b0f), Row(s002,5,41,[D@3d573049), Row(s002,5,42,[D@e150d1d), Row(s002,5,43,[D@3177f2a), Row(s002,5,44,[D@7691df14), Row(s002,5,45,[D@72407cf7), Row(s002,5,46,[D@541bea47), Row(s002,5,47,[D@6c4d21c1), Row(s002,5,48,[D@14dc2a23), Row(s002,5,49,[D@650a4b8b), Row(s002,5,50,[D@6ec8dcbf), Row(s002,6,1,[D@6b0eab8e), Row(s002,6,2,[D@1e7619fe), Row(s002,6,3,[D@29174fa8), Row(s002,6,4,[D@1925311), Row(s002,6,5,[D@1fdeda76), Row(s002,6,6,[D@3149899a), Row(s002,6,7,[D@2230ea14), Row(s002,6,8,[D@31a93f98), Row(s002,6,9,[D@584d4eb1), Row(s002,6,10,[D@2535197b), Row(s002,6,11,[D@f03783), Row(s002,6,12,[D@785d8dfa), Row(s002,6,13,[D@4a577c86), Row(s002,6,14,[D@47244a76), Row(s002,6,15,[D@4669313e), Row(s002,6,16,[D@52cf4654), Row(s002,6,17,[D@238db3c7), Row(s002,6,18,[D@461f2a3a), Row(s002,6,19,[D@49700306), Row(s002,6,20,[D@61a5019a), Row(s002,6,21,[D@2f7885e2), Row(s002,6,22,[D@108934cd), Row(s002,6,23,[D@59a87de9), Row(s002,6,24,[D@10c81e97), Row(s002,6,25,[D@7b74def9), Row(s002,6,26,[D@2282b206), Row(s002,6,27,[D@385fc48a), Row(s002,6,28,[D@683349b0), Row(s002,6,29,[D@35edc159), Row(s002,6,30,[D@2c84760), Row(s002,6,31,[D@44f5b827), Row(s002,6,32,[D@36f917c0), Row(s002,6,33,[D@6dc5ef4f), Row(s002,6,34,[D@2df656d0), Row(s002,6,35,[D@44abf7c9), Row(s002,6,36,[D@3a92bf8b), Row(s002,6,37,[D@786f8001), Row(s002,6,38,[D@6730090a), Row(s002,6,39,[D@7cbebf9a), Row(s002,6,40,[D@53ff3961), Row(s002,6,41,[D@5714d497), Row(s002,6,42,[D@300bef26), Row(s002,6,43,[D@39c0d4d2), Row(s002,6,44,[D@135ed6ba), Row(s002,6,45,[D@629f182a), Row(s002,6,46,[D@d02a980), Row(s002,6,47,[D@325b8ab6), Row(s002,6,48,[D@57d56141), Row(s002,6,49,[D@4d917dd9), Row(s002,6,50,[D@55c79342), Row(s002,7,1,[D@3b27ad76), Row(s002,7,2,[D@47040c3a), Row(s002,7,3,[D@1bdceb6), Row(s002,7,4,[D@68890040), Row(s002,7,5,[D@6e434c3a), Row(s002,7,6,[D@941f814), Row(s002,7,7,[D@66e64ff4), Row(s002,7,8,[D@46cdc868), Row(s002,7,9,[D@329b6e17), Row(s002,7,10,[D@341ca108), Row(s002,7,11,[D@60d1c190), Row(s002,7,12,[D@48f7db6a), Row(s002,7,13,[D@2175efb5), Row(s002,7,14,[D@70301e72), Row(s002,7,15,[D@1e838ca5), Row(s002,7,16,[D@68592894), Row(s002,7,17,[D@66df7a6d), Row(s002,7,18,[D@16ec3b4a), Row(s002,7,19,[D@14086b96), Row(s002,7,20,[D@3534ec0b), Row(s002,7,21,[D@28a5e4d2), Row(s002,7,22,[D@5f64bce7), Row(s002,7,23,[D@8e72846), Row(s002,7,24,[D@1aa1c9e5), Row(s002,7,25,[D@1d253d17), Row(s002,7,26,[D@675c39d0), Row(s002,7,27,[D@56c89865), Row(s002,7,28,[D@42328db8), Row(s002,7,29,[D@76cbdd6c), Row(s002,7,30,[D@705fe8dc), Row(s002,7,31,[D@62c64d02), Row(s002,7,32,[D@34cf53af), Row(s002,7,33,[D@1c47c8e7), Row(s002,7,34,[D@1351cabb), Row(s002,7,35,[D@43af2b8e), Row(s002,7,36,[D@db34a7f), Row(s002,7,37,[D@2f11abde), Row(s002,7,38,[D@3208b895), Row(s002,7,39,[D@8411601), Row(s002,7,40,[D@1fb655c4), Row(s002,7,41,[D@3d5b52b7), Row(s002,7,42,[D@4ae08999), Row(s002,7,43,[D@4a916fda), Row(s002,7,44,[D@67a41b39), Row(s002,7,45,[D@8d2390), Row(s002,7,46,[D@4e6fce59), Row(s002,7,47,[D@fc197f1), Row(s002,7,48,[D@487b38e3), Row(s002,7,49,[D@218313df), Row(s002,7,50,[D@11ae1cb0), Row(s002,8,1,[D@12d36f6b), Row(s002,8,2,[D@37ee1f2), Row(s002,8,3,[D@3ada07a9), Row(s002,8,4,[D@5b70d7de), Row(s002,8,5,[D@52d153ec), Row(s002,8,6,[D@26d44935), Row(s002,8,7,[D@4cd9f509), Row(s002,8,8,[D@114a156b), Row(s002,8,9,[D@495c6a9d), Row(s002,8,10,[D@4dc51f62), Row(s002,8,11,[D@4e37da6e), Row(s002,8,12,[D@f976f8e), Row(s002,8,13,[D@2535f463), Row(s002,8,14,[D@406ec1a8), Row(s002,8,15,[D@30fa8761), Row(s002,8,16,[D@42575ef), Row(s002,8,17,[D@e390fe4), Row(s002,8,18,[D@386cfc0a), Row(s002,8,19,[D@5c49a5e9), Row(s002,8,20,[D@73ec2982), Row(s002,8,21,[D@35ec4e34), Row(s002,8,22,[D@6abf682f), Row(s002,8,23,[D@7bc8fe7c), Row(s002,8,24,[D@697a78be), Row(s002,8,25,[D@3eb0164b), Row(s002,8,26,[D@2f5fefa2), Row(s002,8,27,[D@13d8df2b), Row(s002,8,28,[D@295dea66), Row(s002,8,29,[D@1761a348), Row(s002,8,30,[D@47137cf9), Row(s002,8,31,[D@12e7d2b7), Row(s002,8,32,[D@542f1457), Row(s002,8,33,[D@4e4e8679), Row(s002,8,34,[D@1266cffd), Row(s002,8,35,[D@3eb8c56c), Row(s002,8,36,[D@1219e340), Row(s002,8,37,[D@285ed234), Row(s002,8,38,[D@ce3e9e5), Row(s002,8,39,[D@7488ceb0), Row(s002,8,40,[D@29562082), Row(s002,8,41,[D@774788ee), Row(s002,8,42,[D@6478eb22), Row(s002,8,43,[D@56b74453), Row(s002,8,44,[D@4efd3c37), Row(s002,8,45,[D@5b6ddfe), Row(s002,8,46,[D@26b41791), Row(s002,8,47,[D@4a4d4ac8), Row(s002,8,48,[D@6d76cb32), Row(s002,8,49,[D@5e1ca24f), Row(s002,8,50,[D@58122e82), Row(s003,1,1,[D@78a92442), Row(s003,1,2,[D@23dd5f3e), Row(s003,1,3,[D@18e88fd0), Row(s003,1,4,[D@51c7d471), Row(s003,1,5,[D@607d613f), Row(s003,1,6,[D@2990e6a1), Row(s003,1,7,[D@755a6a4c), Row(s003,1,8,[D@1a514cca), Row(s003,1,9,[D@11aeb226), Row(s003,1,10,[D@3f25fb5c), Row(s003,1,11,[D@190bfefe), Row(s003,1,12,[D@92cd609), Row(s003,1,13,[D@6d776a88), Row(s003,1,14,[D@7d988361), Row(s003,1,15,[D@3b227e22), Row(s003,1,16,[D@54d17bd1), Row(s003,1,17,[D@22478e9), Row(s003,1,18,[D@3b1e6047), Row(s003,1,19,[D@13e5da68), Row(s003,1,20,[D@4c352436), Row(s003,1,21,[D@6d77ee69), Row(s003,1,22,[D@25a3a7d8), Row(s003,1,23,[D@18a81f9e), Row(s003,1,24,[D@7dd98c29), Row(s003,1,25,[D@2f0f315d), Row(s003,1,26,[D@172ace03), Row(s003,1,27,[D@4fa66797), Row(s003,1,28,[D@7e2f128e), Row(s003,1,29,[D@28fc41cf), Row(s003,1,30,[D@6967c805), Row(s003,1,31,[D@15819061), Row(s003,1,32,[D@133c5b3d), Row(s003,1,33,[D@590482ac), Row(s003,1,34,[D@ef44e69), Row(s003,1,35,[D@176fc54e), Row(s003,1,36,[D@66fb912d), Row(s003,1,37,[D@1b976ebc), Row(s003,1,38,[D@373ceca1), Row(s003,1,39,[D@5e101abd), Row(s003,1,40,[D@64d89aab), Row(s003,1,41,[D@449aea02), Row(s003,1,42,[D@14135fd4), Row(s003,1,43,[D@4a087a19), Row(s003,1,44,[D@6a25bc31), Row(s003,1,45,[D@797c818d), Row(s003,1,46,[D@779f8c89), Row(s003,1,47,[D@7e4ef8d1), Row(s003,1,48,[D@39cd171d), Row(s003,1,49,[D@24a09940), Row(s003,1,50,[D@2f50b299), Row(s003,2,1,[D@26515fd2), Row(s003,2,2,[D@77f5d1fa), Row(s003,2,3,[D@573f3cdd), Row(s003,2,4,[D@7dd188d9), Row(s003,2,5,[D@51d2e77e), Row(s003,2,6,[D@8f186bf), Row(s003,2,7,[D@26869aa8), Row(s003,2,8,[D@57e049e1), Row(s003,2,9,[D@114fad74), Row(s003,2,10,[D@158f059c), Row(s003,2,11,[D@7ce9e5f), Row(s003,2,12,[D@52b46006), Row(s003,2,13,[D@3e7c4329), Row(s003,2,14,[D@5332129f), Row(s003,2,15,[D@20fd42c0), Row(s003,2,16,[D@51b8b289), Row(s003,2,17,[D@d81d69c), Row(s003,2,18,[D@4ee49b59), Row(s003,2,19,[D@4452b07), Row(s003,2,20,[D@10fdedfc), Row(s003,2,21,[D@13cbec49), Row(s003,2,22,[D@791f833a), Row(s003,2,23,[D@54af920d), Row(s003,2,24,[D@2bc20769), Row(s003,2,25,[D@67271ffc), Row(s003,2,26,[D@62245671), Row(s003,2,27,[D@4a338fc2), Row(s003,2,28,[D@717120a2), Row(s003,2,29,[D@2e76098f), Row(s003,2,30,[D@6e2155ee), Row(s003,2,31,[D@383a8a77), Row(s003,2,32,[D@40b6c9e2), Row(s003,2,33,[D@5e929a0a), Row(s003,2,34,[D@3a782a13), Row(s003,2,35,[D@56fd6619), Row(s003,2,36,[D@20724cfd), Row(s003,2,37,[D@6a7ac033), Row(s003,2,38,[D@112957dd), Row(s003,2,39,[D@2c59364f), Row(s003,2,40,[D@1efe929d), Row(s003,2,41,[D@2239b229), Row(s003,2,42,[D@79f58e0c), Row(s003,2,43,[D@1c7b3433), Row(s003,2,44,[D@76fb375b), Row(s003,2,45,[D@193c6b57), Row(s003,2,46,[D@4cec0392), Row(s003,2,47,[D@9737c90), Row(s003,2,48,[D@269dd30a), Row(s003,2,49,[D@5c01725d), Row(s003,2,50,[D@70dd1adc), Row(s003,3,1,[D@62d87fab), Row(s003,3,2,[D@2a95e579), Row(s003,3,3,[D@7d51f9ec), Row(s003,3,4,[D@65c21760), Row(s003,3,5,[D@44c61954), Row(s003,3,6,[D@417d8298), Row(s003,3,7,[D@33918dc2), Row(s003,3,8,[D@469de5c7), Row(s003,3,9,[D@32e558f9), Row(s003,3,10,[D@1f21757f), Row(s003,3,11,[D@206104c4), Row(s003,3,12,[D@97b6ed2), Row(s003,3,13,[D@1141cd94), Row(s003,3,14,[D@55fd84e), Row(s003,3,15,[D@2d30bb05), Row(s003,3,16,[D@7f6f4d8f), Row(s003,3,17,[D@60dd029b), Row(s003,3,18,[D@1bbb2b66), Row(s003,3,19,[D@33fb5387), Row(s003,3,20,[D@36fd7342), Row(s003,3,21,[D@3e3c76dc), Row(s003,3,22,[D@7c1e9a66), Row(s003,3,23,[D@15109909), Row(s003,3,24,[D@482a9f8a), Row(s003,3,25,[D@15fd8ac5), Row(s003,3,26,[D@1db8efb6), Row(s003,3,27,[D@cf1f5d9), Row(s003,3,28,[D@103bed02), Row(s003,3,29,[D@6969e662), Row(s003,3,30,[D@33f661a6), Row(s003,3,31,[D@302a153c), Row(s003,3,32,[D@7fb6bdc6), Row(s003,3,33,[D@594a0ea4), Row(s003,3,34,[D@59b1af7a), Row(s003,3,35,[D@3952d285), Row(s003,3,36,[D@734000e4), Row(s003,3,37,[D@7a761f06), Row(s003,3,38,[D@2e68a54d), Row(s003,3,39,[D@1018cff), Row(s003,3,40,[D@7235eb1b), Row(s003,3,41,[D@38714474), Row(s003,3,42,[D@53d8dcfa), Row(s003,3,43,[D@5eb3c40a), Row(s003,3,44,[D@30391f4), Row(s003,3,45,[D@31633704), Row(s003,3,46,[D@24c912de), Row(s003,3,47,[D@649a11d9), Row(s003,3,48,[D@7b89b08f), Row(s003,3,49,[D@53fa73ed), Row(s003,3,50,[D@3fc9d4ae), Row(s003,4,1,[D@be90e57), Row(s003,4,2,[D@3dd2da6d), Row(s003,4,3,[D@3db7b321), Row(s003,4,4,[D@4c2a6c9d), Row(s003,4,5,[D@f7248f9), Row(s003,4,6,[D@2458eac8), Row(s003,4,7,[D@24766bd9), Row(s003,4,8,[D@3b27b54e), Row(s003,4,9,[D@260f1753), Row(s003,4,10,[D@4562a7f0), Row(s003,4,11,[D@525d3426), Row(s003,4,12,[D@545666e6), Row(s003,4,13,[D@a3d56b0), Row(s003,4,14,[D@5ab03d20), Row(s003,4,15,[D@6177ce54), Row(s003,4,16,[D@6f1f5ca), Row(s003,4,17,[D@6619bb72), Row(s003,4,18,[D@3d9bc3ac), Row(s003,4,19,[D@6241af25), Row(s003,4,20,[D@6b174902), Row(s003,4,21,[D@40febd39), Row(s003,4,22,[D@239b8029), Row(s003,4,23,[D@4c4c3bf8), Row(s003,4,24,[D@1d42242a), Row(s003,4,25,[D@286055ce), Row(s003,4,26,[D@57051223), Row(s003,4,27,[D@7a3b70c0), Row(s003,4,28,[D@76d478d9), Row(s003,4,29,[D@5cb09de8), Row(s003,4,30,[D@235b1857), Row(s003,4,31,[D@2c7c18c), Row(s003,4,32,[D@570073bd), Row(s003,4,33,[D@f07fb68), Row(s003,4,34,[D@74e5c37f), Row(s003,4,35,[D@4892a7ce), Row(s003,4,36,[D@1c5bb8fa), Row(s003,4,37,[D@2cb7cca2), Row(s003,4,38,[D@76131c70), Row(s003,4,39,[D@2be279ab), Row(s003,4,40,[D@6a3f9845), Row(s003,4,41,[D@78ff8b7c), Row(s003,4,42,[D@1661f88f), Row(s003,4,43,[D@2ef6e4c9), Row(s003,4,44,[D@381dace2), Row(s003,4,45,[D@3cbd64f6), Row(s003,4,46,[D@2581466e), Row(s003,4,47,[D@3cc82ebb), Row(s003,4,48,[D@6967ef7c), Row(s003,4,49,[D@3e2abc72), Row(s003,4,50,[D@11373fef), Row(s003,5,1,[D@6cf76684), Row(s003,5,2,[D@3a3d6869), Row(s003,5,3,[D@519f8a70), Row(s003,5,4,[D@797f0feb), Row(s003,5,5,[D@2eeb8506), Row(s003,5,6,[D@7f44de92), Row(s003,5,7,[D@52250700), Row(s003,5,8,[D@53a45af8), Row(s003,5,9,[D@2115363f), Row(s003,5,10,[D@787cdcc1), Row(s003,5,11,[D@21bc9c9), Row(s003,5,12,[D@739920e8), Row(s003,5,13,[D@7b35546a), Row(s003,5,14,[D@65310519), Row(s003,5,15,[D@39b8ddf7), Row(s003,5,16,[D@31c3448), Row(s003,5,17,[D@52dbb645), Row(s003,5,18,[D@3f2f78ca), Row(s003,5,19,[D@40074dbd), Row(s003,5,20,[D@22db8f81), Row(s003,5,21,[D@2dbd7c01), Row(s003,5,22,[D@6910b854), Row(s003,5,23,[D@13007a6e), Row(s003,5,24,[D@6d595808), Row(s003,5,25,[D@2bc26cd6), Row(s003,5,26,[D@47fca3e2), Row(s003,5,27,[D@573f6279), Row(s003,5,28,[D@7081f98e), Row(s003,5,29,[D@481d9f94), Row(s003,5,30,[D@6adcd6c6), Row(s003,5,31,[D@46dc4d4e), Row(s003,5,32,[D@396e9192), Row(s003,5,33,[D@1dab4814), Row(s003,5,34,[D@11cdc781), Row(s003,5,35,[D@355f4ecb), Row(s003,5,36,[D@78f0abf3), Row(s003,5,37,[D@3f5ca711), Row(s003,5,38,[D@40d29eb4), Row(s003,5,39,[D@f34a973), Row(s003,5,40,[D@72662455), Row(s003,5,41,[D@28d86127), Row(s003,5,42,[D@7c2b6db6), Row(s003,5,43,[D@567e2c71), Row(s003,5,44,[D@1579ee67), Row(s003,5,45,[D@7ec364b6), Row(s003,5,46,[D@59a2f005), Row(s003,5,47,[D@7e1842e4), Row(s003,5,48,[D@24c88196), Row(s003,5,49,[D@414bb76d), Row(s003,5,50,[D@f274599), Row(s003,6,1,[D@331429fb), Row(s003,6,2,[D@53b0da3e), Row(s003,6,3,[D@4f5cfffa), Row(s003,6,4,[D@7a627005), Row(s003,6,5,[D@68abd543), Row(s003,6,6,[D@3d1f8342), Row(s003,6,7,[D@15140834), Row(s003,6,8,[D@7c9fa0cb), Row(s003,6,9,[D@4a2873d6), Row(s003,6,10,[D@becec42), Row(s003,6,11,[D@3e8c00a3), Row(s003,6,12,[D@3f147641), Row(s003,6,13,[D@36ab14b6), Row(s003,6,14,[D@5ac970dd), Row(s003,6,15,[D@49eea3f), Row(s003,6,16,[D@18242293), Row(s003,6,17,[D@76c49b85), Row(s003,6,18,[D@679a4218), Row(s003,6,19,[D@142693c6), Row(s003,6,20,[D@2daf1b6b), Row(s003,6,21,[D@7fe5a5e8), Row(s003,6,22,[D@4adab28e), Row(s003,6,23,[D@6a42a0b0), Row(s003,6,24,[D@3fe39ad0), Row(s003,6,25,[D@6dfba221), Row(s003,6,26,[D@722a23d2), Row(s003,6,27,[D@d125a07), Row(s003,6,28,[D@2e84646f), Row(s003,6,29,[D@1ede2134), Row(s003,6,30,[D@3d49b58e), Row(s003,6,31,[D@22941242), Row(s003,6,32,[D@2f3ead63), Row(s003,6,33,[D@4086fe31), Row(s003,6,34,[D@3013d66a), Row(s003,6,35,[D@3297d428), Row(s003,6,36,[D@681822ac), Row(s003,6,37,[D@1f182ee8), Row(s003,6,38,[D@31160be7), Row(s003,6,39,[D@3d2cbf79), Row(s003,6,40,[D@1488e732), Row(s003,6,41,[D@4a39e425), Row(s003,6,42,[D@4b7187b6), Row(s003,6,43,[D@137e3ed6), Row(s003,6,44,[D@409cfafc), Row(s003,6,45,[D@45812606), Row(s003,6,46,[D@28a5537), Row(s003,6,47,[D@60e0533e), Row(s003,6,48,[D@470c1ec4), Row(s003,6,49,[D@bf1b135), Row(s003,6,50,[D@5b827e91), Row(s003,7,1,[D@3919af7c), Row(s003,7,2,[D@1ec47ca5), Row(s003,7,3,[D@183a0e51), Row(s003,7,4,[D@50048d31), Row(s003,7,5,[D@2412aa02), Row(s003,7,6,[D@198edb71), Row(s003,7,7,[D@510e0697), Row(s003,7,8,[D@25176482), Row(s003,7,9,[D@14e49898), Row(s003,7,10,[D@7b5e8c26), Row(s003,7,11,[D@5a450764), Row(s003,7,12,[D@44e84bda), Row(s003,7,13,[D@74782b87), Row(s003,7,14,[D@7bc8a692), Row(s003,7,15,[D@9c4e0a8), Row(s003,7,16,[D@ff4dcd1), Row(s003,7,17,[D@3a65eabb), Row(s003,7,18,[D@4273753), Row(s003,7,19,[D@2a4856df), Row(s003,7,20,[D@3f30513), Row(s003,7,21,[D@165607e4), Row(s003,7,22,[D@2bf627d2), Row(s003,7,23,[D@43e062dd), Row(s003,7,24,[D@58a02c2f), Row(s003,7,25,[D@7eef79f8), Row(s003,7,26,[D@643d1940), Row(s003,7,27,[D@248b7990), Row(s003,7,28,[D@7d93f87a), Row(s003,7,29,[D@78366e89), Row(s003,7,30,[D@74cd9fd6), Row(s003,7,31,[D@b753f26), Row(s003,7,32,[D@69c7461a), Row(s003,7,33,[D@22ce3f8d), Row(s003,7,34,[D@3a65372d), Row(s003,7,35,[D@184bb348), Row(s003,7,36,[D@4b6fc1cd), Row(s003,7,37,[D@188ead7a), Row(s003,7,38,[D@b413d19), Row(s003,7,39,[D@4e550eca), Row(s003,7,40,[D@7b01cf64), Row(s003,7,41,[D@16095803), Row(s003,7,42,[D@1422162e), Row(s003,7,43,[D@72e7693e), Row(s003,7,44,[D@7e8e2e9), Row(s003,7,45,[D@5bfd7b57), Row(s003,7,46,[D@5f6a8560), Row(s003,7,47,[D@160db92a), Row(s003,7,48,[D@5632fea8), Row(s003,7,49,[D@66a510fa), Row(s003,7,50,[D@6defc8cb), Row(s003,8,1,[D@16d0f8b5), Row(s003,8,2,[D@57d68379), Row(s003,8,3,[D@193a7bb9), Row(s003,8,4,[D@a0041c5), Row(s003,8,5,[D@1b841560), Row(s003,8,6,[D@782a9022), Row(s003,8,7,[D@3207cb2d), Row(s003,8,8,[D@3a81bac1), Row(s003,8,9,[D@19587e4), Row(s003,8,10,[D@2d12ac74), Row(s003,8,11,[D@21c05458), Row(s003,8,12,[D@16a0a513), Row(s003,8,13,[D@3ba7ba84), Row(s003,8,14,[D@36ec088), Row(s003,8,15,[D@20af2629), Row(s003,8,16,[D@33b49712), Row(s003,8,17,[D@35c1687a), Row(s003,8,18,[D@405e94ca), Row(s003,8,19,[D@19996c86), Row(s003,8,20,[D@e8264a0), Row(s003,8,21,[D@30be5fb2), Row(s003,8,22,[D@4f275ab), Row(s003,8,23,[D@56ddd4ef), Row(s003,8,24,[D@4b670df0), Row(s003,8,25,[D@96698e1), Row(s003,8,26,[D@1eaefb4b), Row(s003,8,27,[D@26ec2edd), Row(s003,8,28,[D@5517af7d), Row(s003,8,29,[D@680b84ae), Row(s003,8,30,[D@1165e47), Row(s003,8,31,[D@46cb027e), Row(s003,8,32,[D@2ecf219d), Row(s003,8,33,[D@1ad5fe1e), Row(s003,8,34,[D@29827f65), Row(s003,8,35,[D@374440d9), Row(s003,8,36,[D@60d05c65), Row(s003,8,37,[D@55406b6f), Row(s003,8,38,[D@6f834ff5), Row(s003,8,39,[D@7a549854), Row(s003,8,40,[D@1805d00f), Row(s003,8,41,[D@4e48db73), Row(s003,8,42,[D@3b41c9a8), Row(s003,8,43,[D@650970ac), Row(s003,8,44,[D@53ba51aa), Row(s003,8,45,[D@5b218bed), Row(s003,8,46,[D@6e9805a8), Row(s003,8,47,[D@403a84c7), Row(s003,8,48,[D@418caa6b), Row(s003,8,49,[D@16a53f54), Row(s003,8,50,[D@383ecd6d), Row(s004,1,1,[D@2c366a11), Row(s004,1,2,[D@8cd420e), Row(s004,1,3,[D@37ad83dc), Row(s004,1,4,[D@793675e1), Row(s004,1,5,[D@66cfee34), Row(s004,1,6,[D@4700dd1), Row(s004,1,7,[D@5f98dde0), Row(s004,1,8,[D@154b328f), Row(s004,1,9,[D@cecc05c), Row(s004,1,10,[D@8765a95), Row(s004,1,11,[D@1198e1a6), Row(s004,1,12,[D@5d0b7650), Row(s004,1,13,[D@378fb38d), Row(s004,1,14,[D@d17e51b), Row(s004,1,15,[D@5b54a0ce), Row(s004,1,16,[D@5debf402), Row(s004,1,17,[D@17b227e9), Row(s004,1,18,[D@253f3739), Row(s004,1,19,[D@5b13b180), Row(s004,1,20,[D@59da1504), Row(s004,1,21,[D@5f51ecb9), Row(s004,1,22,[D@30b8e95), Row(s004,1,23,[D@4552a0c5), Row(s004,1,24,[D@4c29ff5e), Row(s004,1,25,[D@1c4df646), Row(s004,1,26,[D@436dbc7c), Row(s004,1,27,[D@1369785c), Row(s004,1,28,[D@1039b660), Row(s004,1,29,[D@63358de7), Row(s004,1,30,[D@4d1543a1), Row(s004,1,31,[D@156679c7), Row(s004,1,32,[D@483157bd), Row(s004,1,33,[D@724b1e9), Row(s004,1,34,[D@60cbf2e7), Row(s004,1,35,[D@46c50778), Row(s004,1,36,[D@48b3ca2), Row(s004,1,37,[D@26027e23), Row(s004,1,38,[D@19e1fcce), Row(s004,1,39,[D@7771c64d), Row(s004,1,40,[D@2ac28a2d), Row(s004,1,41,[D@1f840a30), Row(s004,1,42,[D@9958282), Row(s004,1,43,[D@702f6e53), Row(s004,1,44,[D@4e020199), Row(s004,1,45,[D@71e857e3), Row(s004,1,46,[D@54cc4ace), Row(s004,1,47,[D@5f1afbf2), Row(s004,1,48,[D@14a3741), Row(s004,1,49,[D@322e2fc4), Row(s004,1,50,[D@4028975), Row(s004,2,1,[D@c4f376c), Row(s004,2,2,[D@5ceff99b), Row(s004,2,3,[D@1ffcadcd), Row(s004,2,4,[D@f25c666), Row(s004,2,5,[D@7aa474b9), Row(s004,2,6,[D@59a46957), Row(s004,2,7,[D@23cc256b), Row(s004,2,8,[D@27bc182), Row(s004,2,9,[D@5ba36cc8), Row(s004,2,10,[D@21b7583a), Row(s004,2,11,[D@6312d61a), Row(s004,2,12,[D@3f396c2b), Row(s004,2,13,[D@7fbc9228), Row(s004,2,14,[D@64d1736d), Row(s004,2,15,[D@1106cbeb), Row(s004,2,16,[D@652ab5d4), Row(s004,2,17,[D@7e1c568b), Row(s004,2,18,[D@1139183e), Row(s004,2,19,[D@36c7c061), Row(s004,2,20,[D@6f35778), Row(s004,2,21,[D@1a47e123), Row(s004,2,22,[D@43e6e2bd), Row(s004,2,23,[D@4b2af668), Row(s004,2,24,[D@577e30e2), Row(s004,2,25,[D@72959dd7), Row(s004,2,26,[D@6926a32), Row(s004,2,27,[D@1a97553e), Row(s004,2,28,[D@3c488fae), Row(s004,2,29,[D@62edd6d5), Row(s004,2,30,[D@773bf340), Row(s004,2,31,[D@57a5763c), Row(s004,2,32,[D@2fe8b699), Row(s004,2,33,[D@233f96cf), Row(s004,2,34,[D@bb6d01b), Row(s004,2,35,[D@77de43c7), Row(s004,2,36,[D@1de87fdb), Row(s004,2,37,[D@423e0b47), Row(s004,2,38,[D@7f352d13), Row(s004,2,39,[D@7af08949), Row(s004,2,40,[D@2438276b), Row(s004,2,41,[D@166e7498), Row(s004,2,42,[D@40659ef3), Row(s004,2,43,[D@3ea1ed77), Row(s004,2,44,[D@5b4796b7), Row(s004,2,45,[D@3ee8f3f3), Row(s004,2,46,[D@521670db), Row(s004,2,47,[D@63edf5bb), Row(s004,2,48,[D@4f8355f), Row(s004,2,49,[D@7d762958), Row(s004,2,50,[D@1c870e85), Row(s004,3,1,[D@104b7083), Row(s004,3,2,[D@55dced18), Row(s004,3,3,[D@19ac2212), Row(s004,3,4,[D@3dfbe484), Row(s004,3,5,[D@767f44d0), Row(s004,3,6,[D@44f9d32a), Row(s004,3,7,[D@3cbdd515), Row(s004,3,8,[D@5e80d9c2), Row(s004,3,9,[D@52554f06), Row(s004,3,10,[D@58bfb6e5), Row(s004,3,11,[D@af8d59a), Row(s004,3,12,[D@52ee53ce), Row(s004,3,13,[D@2abb0bea), Row(s004,3,14,[D@f1698c6), Row(s004,3,15,[D@438ed8bb), Row(s004,3,16,[D@63de8327), Row(s004,3,17,[D@11c830ed), Row(s004,3,18,[D@2a235aba), Row(s004,3,19,[D@1f5d0445), Row(s004,3,20,[D@80d6b32), Row(s004,3,21,[D@58927d86), Row(s004,3,22,[D@68d41aa4), Row(s004,3,23,[D@1fdc44d7), Row(s004,3,24,[D@7cebe8e5), Row(s004,3,25,[D@37ded4b3), Row(s004,3,26,[D@7f17e9d6), Row(s004,3,27,[D@210f01f), Row(s004,3,28,[D@219f9c78), Row(s004,3,29,[D@6025afb4), Row(s004,3,30,[D@203cb33f), Row(s004,3,31,[D@25293f2f), Row(s004,3,32,[D@78881bae), Row(s004,3,33,[D@351d5304), Row(s004,3,34,[D@70fdbbd3), Row(s004,3,35,[D@1c4122a4), Row(s004,3,36,[D@24ac1fe9), Row(s004,3,37,[D@7bf6fd0b), Row(s004,3,38,[D@6648f285), Row(s004,3,39,[D@7309b8ea), Row(s004,3,40,[D@379ea235), Row(s004,3,41,[D@7b4c1f68), Row(s004,3,42,[D@5a31065e), Row(s004,3,43,[D@64412b1a), Row(s004,3,44,[D@260cb2ad), Row(s004,3,45,[D@3d205e5b), Row(s004,3,46,[D@6ff1bc57), Row(s004,3,47,[D@2055348), Row(s004,3,48,[D@41df007f), Row(s004,3,49,[D@7eb24c99), Row(s004,3,50,[D@1c43541c), Row(s004,4,1,[D@3474cbcf), Row(s004,4,2,[D@d91e9c6), Row(s004,4,3,[D@61abba69), Row(s004,4,4,[D@67cef1f4), Row(s004,4,5,[D@75766471), Row(s004,4,6,[D@772b7cc0), Row(s004,4,7,[D@4beff8be), Row(s004,4,8,[D@5bbef166), Row(s004,4,9,[D@1dad538c), Row(s004,4,10,[D@314cf185), Row(s004,4,11,[D@5d2c51a), Row(s004,4,12,[D@29cb3107), Row(s004,4,13,[D@5e0d3681), Row(s004,4,14,[D@81b341c), Row(s004,4,15,[D@1b72da10), Row(s004,4,16,[D@6b418370), Row(s004,4,17,[D@5c4f19a7), Row(s004,4,18,[D@d257de6), Row(s004,4,19,[D@8a9408), Row(s004,4,20,[D@6737dd6a), Row(s004,4,21,[D@4391720a), Row(s004,4,22,[D@65fdfdd3), Row(s004,4,23,[D@31031fb0), Row(s004,4,24,[D@68064877), Row(s004,4,25,[D@20cf765f), Row(s004,4,26,[D@2ad604f0), Row(s004,4,27,[D@3016085), Row(s004,4,28,[D@599ed562), Row(s004,4,29,[D@2b83d80), Row(s004,4,30,[D@18d358a3), Row(s004,4,31,[D@10de1674), Row(s004,4,32,[D@3fc4e4c8), Row(s004,4,33,[D@7cd38a8d), Row(s004,4,34,[D@7e47d3f4), Row(s004,4,35,[D@1eca17fe), Row(s004,4,36,[D@630524b), Row(s004,4,37,[D@665737e2), Row(s004,4,38,[D@264f80af), Row(s004,4,39,[D@6874167f), Row(s004,4,40,[D@6c52b330), Row(s004,4,41,[D@33e5617f), Row(s004,4,42,[D@69f5c554), Row(s004,4,43,[D@21faf1fb), Row(s004,4,44,[D@58480dc7), Row(s004,4,45,[D@403e7128), Row(s004,4,46,[D@7a6d31e), Row(s004,4,47,[D@71259f38), Row(s004,4,48,[D@691b82ee), Row(s004,4,49,[D@5a9f09d4), Row(s004,4,50,[D@6b101fba))\ngetTimingsFactors: (data: Array[Row])Array[Double]\ngetDistance: (array1: Array[Double], array2: Array[Double], timingFactors: Array[Double])Double\ngetAverageTimings: (data: Array[Row])Array[Double]\ngetRepresentativeSet: (data: Array[Row], allowedFalseNegativeRate: Double)Array[Row]\nsimulateCreditabilityScore: (data: Array[Row], averageTimings: Array[Double], timingFactors: Array[Double], threshold: Double)Boolean\nanalyseSubject: (data: Array[Row], subject: String)Result\nsubjects: Array[String] = Array(s002, s003, s004, s005, s007, s008, s010, s011, s012, s013, s015, s016, s017, s018, s019, s020, s021, s022, s024, s025, s026, s027, s028, s029, s030, s031, s032, s033, s034, s035, s036, s037, s038, s039, s040, s041, s042, s043, s044, s046, s047, s048, s049, s050, s051, s052, s053, s054, s055, s056, s057)\nerrorRates: Array[Result] = Array(Result(s020,1.2773733159529799,0.15,0.598,0.125,0.4525,0.3852353878543283), Result(s049,1.5610245797249591,0.175,0.34025,0.125,0.34,0.3793239190307475), Result(s040,1.6911959491290878,0.2,0.2587,0.125,0.2225,0.316363916748968), Result(s003,1.0476080398348857,0.1875,0.22,0.0,0.075,0.2986876829253832), Result(s011,0.9377496093750001,0.1875,0.095,0.125,0.03,0.2979422510768676), Result(s016,1.6007095971490837,0.1,0.06355,0.125,0.055,0.29315699116875016), Result(s047,1.3243246111732865,0.225,0.23255,0.25,0.18,0.2854641616364273), Result(s035,1.102367579807221,0.0875,0.31445,0.125,0.2175,0.2828022648152238), Result(s033,1.5676547712364537,0.1625,0.06355,0.125,0.0675,0.27654056841782493), Result(s057,0.8799113281250006,0.15,0.23825,0.0,0.16,0.272443507449229), Result(s041,1.0125387388192428,0.125,0.0624,0.375,0.0225,0.2689758222952891), Result(s032,0.8967103073605761,0.2875,0.3911,0.125,0.2725,0.26868391954648063), Result(s031,1.1132214018404674,0.2375,0.2959,0.125,0.215,0.26591019817145756), Result(s051,0.8502374999999995,0.2125,0.2117,0.125,0.1475,0.26206643792024564), Result(s034,0.8844475056534284,0.25,0.21155,0.125,0.165,0.26021019814382396), Result(s015,0.8093712335643646,0.3,0.1392,0.125,0.14,0.256853144261177), Result(s002,0.963913928310338,0.175,0.2981,0.25,0.175,0.2533316602779885), Result(s018,0.9793204306081632,0.1625,0.18535,0.125,0.0925,0.2512053325367009), Result(s022,1.3952974947277794,0.1625,0.0169,0.0,0.0025,0.250629044702529), Result(s036,1.4926723057428783,0.15,0.0012,0.125,0.0,0.24866315657168128), Result(s007,0.755808657541738,0.2,0.17755,0.25,0.1225,0.24529621582479325), Result(s012,0.960161167580198,0.1375,0.06505,0.0,0.0225,0.24133987216822197), Result(s056,0.8017226562499999,0.2375,0.12645,0.0,0.0625,0.2394794206689195), Result(s052,1.137289883439248,0.2125,0.0028,0.125,0.0,0.23897403575537088), Result(s046,1.0582540745489628,0.2,0.1334,0.125,0.08,0.2365657561461832), Result(s008,0.7613779613559349,0.1875,0.12785,0.125,0.1075,0.23029609471251), Result(s043,1.177606191023883,0.1125,0.0142,0.125,0.005,0.229758574811945), Result(s027,1.0010160577417553,0.1,0.0963,0.125,0.045,0.2271888194045635), Result(s037,0.7253005709021437,0.2625,0.16415,0.125,0.0825,0.2239235268864702), Result(s004,0.8135658544446964,0.1875,0.11995,0.0,0.065,0.219099270263811), Result(s053,0.7178906249999999,0.15,0.0288,0.125,0.0175,0.21528099100945902), Result(s030,1.1121031525739422,0.175,0.0208,0.0,0.015,0.2130951480275123), Result(s050,0.8208762328522515,0.1875,0.1148,0.125,0.0525,0.20264523964437478), Result(s055,0.5433457031250001,0.25,0.0024,0.125,0.0,0.2003767927583647), Result(s021,0.7669687824250512,0.125,0.0602,0.125,0.0175,0.19720745566079378), Result(s013,0.5741658913580499,0.2375,0.03765,0.125,0.035,0.19346059746926916), Result(s038,0.7296060784308096,0.075,0.0149,0.0,0.005,0.19278415330516857), Result(s029,0.6458359950536199,0.15,0.05245,0.125,0.02,0.19252619318320283), Result(s044,0.7764086185062573,0.175,0.0126,0.125,0.0025,0.19194991790272226), Result(s048,0.671234040836241,0.15,0.0456,0.0,0.025,0.19097978977656802), Result(s026,0.7262589206744209,0.2,0.09255,0.0,0.0425,0.1882074017237553), Result(s024,0.8573151296497107,0.1375,0.017,0.125,0.005,0.18723405877440852), Result(s054,0.6671500241631287,0.1625,0.04825,0.0,0.0225,0.18250062550883453), Result(s028,0.7346201748924511,0.1875,0.0203,0.0,0.0075,0.17742233160862106), Result(s005,0.9843046874999992,0.075,0.01725,0.0,0.005,0.17449663128194642), Result(s010,0.5444652343749998,0.2625,0.00495,0.0,0.0,0.1647376199855452), Result(s039,0.5134987251183448,0.175,0.0136,0.25,0.0,0.15926721468697938), Result(s019,0.7057269855505732,0.125,3.5E-4,0.0,0.0,0.15855657596806447), Result(s017,0.5358350346037614,0.1875,0.00475,0.125,0.005,0.152714215390689), Result(s025,0.6800326408152348,0.275,0.0065,0.125,0.005,0.14918255906996006), Result(s042,0.5834686846173982,0.15,1.5E-4,0.125,0.0,0.14209711922047263))\nerrorRatesForConsistent: Array[Result] = Array(Result(s020,1.2773733159529799,0.15,0.598,0.125,0.4525,0.3852353878543283), Result(s049,1.5610245797249591,0.175,0.34025,0.125,0.34,0.3793239190307475), Result(s040,1.6911959491290878,0.2,0.2587,0.125,0.2225,0.316363916748968), Result(s003,1.0476080398348857,0.1875,0.22,0.0,0.075,0.2986876829253832), Result(s011,0.9377496093750001,0.1875,0.095,0.125,0.03,0.2979422510768676), Result(s016,1.6007095971490837,0.1,0.06355,0.125,0.055,0.29315699116875016), Result(s047,1.3243246111732865,0.225,0.23255,0.25,0.18,0.2854641616364273), Result(s035,1.102367579807221,0.0875,0.31445,0.125,0.2175,0.2828022648152238), Result(s033,1.5676547712364537,0.1625,0.06355,0.125,0.0675,0.27654056841782493), Result(s057,0.8799113281250006,0.15,0.23825,0.0,0.16,0.272443507449229), Result(s041,1.0125387388192428,0.125,0.0624,0.375,0.0225,0.2689758222952891), Result(s032,0.8967103073605761,0.2875,0.3911,0.125,0.2725,0.26868391954648063), Result(s031,1.1132214018404674,0.2375,0.2959,0.125,0.215,0.26591019817145756), Result(s051,0.8502374999999995,0.2125,0.2117,0.125,0.1475,0.26206643792024564), Result(s034,0.8844475056534284,0.25,0.21155,0.125,0.165,0.26021019814382396), Result(s015,0.8093712335643646,0.3,0.1392,0.125,0.14,0.256853144261177), Result(s002,0.963913928310338,0.175,0.2981,0.25,0.175,0.2533316602779885), Result(s018,0.9793204306081632,0.1625,0.18535,0.125,0.0925,0.2512053325367009), Result(s022,1.3952974947277794,0.1625,0.0169,0.0,0.0025,0.250629044702529), Result(s036,1.4926723057428783,0.15,0.0012,0.125,0.0,0.24866315657168128), Result(s007,0.755808657541738,0.2,0.17755,0.25,0.1225,0.24529621582479325), Result(s012,0.960161167580198,0.1375,0.06505,0.0,0.0225,0.24133987216822197), Result(s056,0.8017226562499999,0.2375,0.12645,0.0,0.0625,0.2394794206689195), Result(s052,1.137289883439248,0.2125,0.0028,0.125,0.0,0.23897403575537088), Result(s046,1.0582540745489628,0.2,0.1334,0.125,0.08,0.2365657561461832), Result(s008,0.7613779613559349,0.1875,0.12785,0.125,0.1075,0.23029609471251), Result(s043,1.177606191023883,0.1125,0.0142,0.125,0.005,0.229758574811945), Result(s027,1.0010160577417553,0.1,0.0963,0.125,0.045,0.2271888194045635), Result(s037,0.7253005709021437,0.2625,0.16415,0.125,0.0825,0.2239235268864702), Result(s004,0.8135658544446964,0.1875,0.11995,0.0,0.065,0.219099270263811), Result(s053,0.7178906249999999,0.15,0.0288,0.125,0.0175,0.21528099100945902), Result(s030,1.1121031525739422,0.175,0.0208,0.0,0.015,0.2130951480275123), Result(s050,0.8208762328522515,0.1875,0.1148,0.125,0.0525,0.20264523964437478), Result(s055,0.5433457031250001,0.25,0.0024,0.125,0.0,0.2003767927583647), Result(s021,0.7669687824250512,0.125,0.0602,0.125,0.0175,0.19720745566079378), Result(s013,0.5741658913580499,0.2375,0.03765,0.125,0.035,0.19346059746926916), Result(s038,0.7296060784308096,0.075,0.0149,0.0,0.005,0.19278415330516857), Result(s029,0.6458359950536199,0.15,0.05245,0.125,0.02,0.19252619318320283), Result(s044,0.7764086185062573,0.175,0.0126,0.125,0.0025,0.19194991790272226), Result(s048,0.671234040836241,0.15,0.0456,0.0,0.025,0.19097978977656802), Result(s026,0.7262589206744209,0.2,0.09255,0.0,0.0425,0.1882074017237553), Result(s024,0.8573151296497107,0.1375,0.017,0.125,0.005,0.18723405877440852), Result(s054,0.6671500241631287,0.1625,0.04825,0.0,0.0225,0.18250062550883453), Result(s028,0.7346201748924511,0.1875,0.0203,0.0,0.0075,0.17742233160862106), Result(s005,0.9843046874999992,0.075,0.01725,0.0,0.005,0.17449663128194642), Result(s010,0.5444652343749998,0.2625,0.00495,0.0,0.0,0.1647376199855452), Result(s039,0.5134987251183448,0.175,0.0136,0.25,0.0,0.15926721468697938), Result(s019,0.7057269855505732,0.125,3.5E-4,0.0,0.0,0.15855657596806447), Result(s017,0.5358350346037614,0.1875,0.00475,0.125,0.005,0.152714215390689), Result(s025,0.6800326408152348,0.275,0.0065,0.125,0.005,0.14918255906996006), Result(s042,0.5834686846173982,0.15,1.5E-4,0.125,0.0,0.14209711922047263))\n</div>","arguments":{},"addedWidgets":{},"removedWidgets":[]},"errorSummary":null,"error":null,"startTime":1.465877746057E12,"submitTime":1.465877546924E12,"finishTime":1.465877757457E12,"collapsed":false,"bindings":{},"inputWidgets":{},"displayType":"table","width":"auto","height":"auto","xColumns":null,"yColumns":null,"pivotColumns":null,"pivotAggregation":null,"customPlotOptions":{},"commentThread":[],"commentsVisible":false,"parentHierarchy":[],"diffInserts":[],"diffDeletes":[],"globalVars":{},"latestUser":"ako60@uclive.ac.nz","commandTitle":"","showCommandTitle":false,"hideCommandCode":false,"hideCommandResult":false,"iPythonMetadata":null,"nuid":"b9a79fbb-819b-4e5a-94a9-3e97ff10b97e"},{"version":"CommandV1","origId":136559,"guid":"4a2e4ba1-b87d-435e-a4b9-9c7d3f131cfd","subtype":"command","commandType":"auto","position":3.0,"command":"%md\n\n# [Scalable Data Science](http://www.math.canterbury.ac.nz/~r.sainudiin/courses/ScalableDataScience/)\n\n## Keystroke Biometric\n### Scalable data science project by [Andrey Konstantinov](https://www.linkedin.com/in/andrey-konstantinov-38234531)\n\n*supported by* [](https://databricks.com/)\nand \n[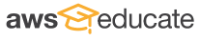](https://www.awseducate.com/microsite/CommunitiesEngageHome)","commandVersion":0,"state":"error","results":null,"errorSummary":null,"error":null,"startTime":0.0,"submitTime":0.0,"finishTime":0.0,"collapsed":false,"bindings":{},"inputWidgets":{},"displayType":"table","width":"auto","height":"auto","xColumns":null,"yColumns":null,"pivotColumns":null,"pivotAggregation":null,"customPlotOptions":{},"commentThread":[],"commentsVisible":false,"parentHierarchy":[],"diffInserts":[],"diffDeletes":[],"globalVars":{},"latestUser":"","commandTitle":"","showCommandTitle":false,"hideCommandCode":false,"hideCommandResult":false,"iPythonMetadata":null,"nuid":"f2a3430b-ea54-4dd8-8147-7c75b6b59d88"}],"dashboards":[],"guid":"3ac3beb7-69c5-409f-a039-878a61d001c3","globalVars":{},"iPythonMetadata":null,"inputWidgets":{}};</script> <script src="https://databricks-prod-cloudfront.cloud.databricks.com/static/201602081754420800-0c2673ac858e227cad536fdb45d140aeded238db/js/notebook-main.js" onerror="window.mainJsLoadError = true;"></script> </head> <body> <script> if (window.mainJsLoadError) { var u = 'https://databricks-prod-cloudfront.cloud.databricks.com/static/201602081754420800-0c2673ac858e227cad536fdb45d140aeded238db/js/notebook-main.js'; var b = document.getElementsByTagName('body')[0]; var c = document.createElement('div'); c.innerHTML = ('<h1>Network Error</h1>' + '<p><b>Please check your network connection and try again.</b></p>' + '<p>Could not load a required resource: ' + u + '</p>'); c.style.margin = '30px'; c.style.padding = '20px 50px'; c.style.backgroundColor = '#f5f5f5'; c.style.borderRadius = '5px'; b.appendChild(c); } </script> </body> </html>