{
"cells": [
{
"cell_type": "markdown",
"metadata": {},
"source": [
"[](https://www.visual-layer.com)"
]
},
{
"cell_type": "markdown",
"metadata": {},
"source": [
"# Image Captioning & Visual Question Answering (VQA) With fastdup\n",
"\n",
"[](https://colab.research.google.com/github/visual-layer/fastdup/blob/main/examples/caption_generation.ipynb)\n",
"[](https://kaggle.com/kernels/welcome?src=https://github.com/visual-layer/fastdup/blob/main/examples/caption_generation.ipynb)\n",
"\n",
"\n",
"This notebook shows how you can use [fastdup](https://github.com/visual-layer/fastdup) to generate image captions. Caption generation has many useful use cases, including zero-shot classification, and accessibility features.\n",
"Additional examples in this notebook include visual question answering (VQA), which can be used for a number of applications such as image retrieval.\n",
"\n",
"The captioning and VQA models employed in this example can generally be run on a CPU, with no GPU needed. The smallest model in this example requires about 0.5s per image caption, allowing 100,000 images to be captioned in half a day."
]
},
{
"cell_type": "markdown",
"metadata": {},
"source": [
"# Install fastdup\n",
"\n",
"First, install fastdup and verify the installation."
]
},
{
"cell_type": "code",
"execution_count": 1,
"metadata": {},
"outputs": [],
"source": [
"!pip install fastdup -Uq"
]
},
{
"cell_type": "markdown",
"metadata": {},
"source": [
"Now, test the installation. If there's no error message, we are ready to go."
]
},
{
"cell_type": "code",
"execution_count": 1,
"metadata": {},
"outputs": [
{
"data": {
"text/plain": [
"'1.39'"
]
},
"execution_count": 1,
"metadata": {},
"output_type": "execute_result"
}
],
"source": [
"import fastdup\n",
"fastdup.__version__"
]
},
{
"cell_type": "markdown",
"metadata": {},
"source": [
"# Load Dataset\n",
"\n",
"In this example we will be using the [COCO Minitrain Dataset](https://github.com/giddyyupp/coco-minitrain), which is a curated mini training set of about 25,000 images (20% of the original COCO dataset).\n",
"We will download the dataset into our local drive."
]
},
{
"cell_type": "code",
"execution_count": null,
"metadata": {},
"outputs": [],
"source": [
"!pip install gdown"
]
},
{
"cell_type": "code",
"execution_count": 3,
"metadata": {},
"outputs": [
{
"name": "stdout",
"output_type": "stream",
"text": [
"Downloading...\n",
"From (uriginal): https://drive.google.com/uc?id=1iSXVTlkV1_DhdYpVDqsjlT4NJFQ7OkyK\n",
"From (redirected): https://drive.google.com/uc?id=1iSXVTlkV1_DhdYpVDqsjlT4NJFQ7OkyK&confirm=t&uuid=8ace7c5d-ec8e-4bba-a8d3-2cb89d555188\n",
"To: /Users/guysinger/Desktop/fastdup/examples/coco_minitrain_25k.zip\n",
"100%|██████████████████████████████████████| 4.90G/4.90G [03:26<00:00, 23.8MB/s]\n",
"Downloading...\n",
"From: https://drive.google.com/uc?id=1i12p23cXlqp1QrXjAD_vu467r4q67Mq9\n",
"To: /Users/guysinger/Desktop/fastdup/examples/coco_minitrain_25k/annotations/coco_minitrain2017.csv\n",
"100%|██████████████████████████████████████| 9.43M/9.43M [00:00<00:00, 12.1MB/s]\n"
]
}
],
"source": [
"# Download coco minitrain dataset.\n",
"!gdown --fuzzy https://drive.google.com/file/d/1iSXVTlkV1_DhdYpVDqsjlT4NJFQ7OkyK/view\n",
"!unzip -qq coco_minitrain_25k.zip\n",
"\n",
"# Download csv annotations\n",
"!cd coco_minitrain_25k/annotations && gdown --fuzzy https://drive.google.com/file/d/1i12p23cXlqp1QrXjAD_vu467r4q67Mq9/view"
]
},
{
"cell_type": "markdown",
"metadata": {},
"source": [
"# Run fastdup\n",
"\n",
"Run fastdup with annotations on the dataset. Here, we set `num_images` to limit the run to 1000 images."
]
},
{
"cell_type": "code",
"execution_count": 8,
"metadata": {},
"outputs": [
{
"name": "stdout",
"output_type": "stream",
"text": [
"Warning: fastdup create() without work_dir argument, output is stored in a folder named work_dir in your current working path.\n",
"FastDup Software, (C) copyright 2022 Dr. Amir Alush and Dr. Danny Bickson.\n",
"2023-09-14 16:39:55 [INFO] Going to loop over dir coco_minitrain_25k\n",
"2023-09-14 16:39:55 [INFO] Found total 1000 images to run on, 1000 train, 0 test, name list 1000, counter 1000 \n",
"2023-09-14 16:39:57 [INFO] Found total 1000 images to run ontimated: 0 Minutes\n",
"2023-09-14 16:39:57 [INFO] 97) Finished write_index() NN model\n",
"2023-09-14 16:39:57 [INFO] Stored nn model index file work_dir/nnf.index\n",
"2023-09-14 16:39:57 [INFO] Total time took 2157 ms\n",
"2023-09-14 16:39:57 [INFO] Found a total of 0 fully identical images (d>0.990), which are 0.00 % of total graph edges\n",
"2023-09-14 16:39:57 [INFO] Found a total of 0 nearly identical images(d>0.980), which are 0.00 % of total graph edges\n",
"2023-09-14 16:39:57 [INFO] Found a total of 0 above threshold images (d>0.900), which are 0.00 % of total graph edges\n",
"2023-09-14 16:39:57 [INFO] Found a total of 100 outlier images (d<0.050), which are 5.00 % of total graph edges\n",
"2023-09-14 16:39:57 [INFO] Min distance found 0.513 max distance 0.894\n",
"2023-09-14 16:39:57 [INFO] Running connected components for ccthreshold 0.900000 \n",
".0\n",
" ########################################################################################\n",
"\n",
"Dataset Analysis Summary: \n",
"\n",
" Dataset contains 1000 images\n",
" Valid images are 100.00% (1,000) of the data, invalid are 0.00% (0) of the data\n",
" Components: failed to find images clustered into components, try to run with lower cc_threshold.\n",
" Outliers: 7.10% (71) of images are possible outliers, and fall in the bottom 5.00% of similarity values.\n",
" For a detailed list of outliers, use `.outliers()`.\n",
"\n",
"########################################################################################\n",
"Would you like to see awesome visualizations for some of the most popular academic datasets?\n",
"Click here to see and learn more: https://app.visual-layer.com/vl-datasets?utm_source=fastdup\n",
"########################################################################################\n"
]
},
{
"name": "stderr",
"output_type": "stream",
"text": [
"/Users/guysinger/Library/Python/3.9/lib/python/site-packages/fastdup/fastdup_controller.py:385: UserWarning: No connected components found, try using a lower threshold\n",
" warnings.warn(f'No connected components found, try using a lower threshold')\n"
]
},
{
"data": {
"text/plain": [
"0"
]
},
"execution_count": 8,
"metadata": {},
"output_type": "execute_result"
}
],
"source": [
"fd = fastdup.create(input_dir='./coco_minitrain_25k')\n",
"fd.run(ccthreshold=0.9, num_images=1000, overwrite=True)"
]
},
{
"cell_type": "markdown",
"metadata": {},
"source": [
"# Generate Captions"
]
},
{
"cell_type": "markdown",
"metadata": {},
"source": [
"fastdup currently supports a number of different captioning and VQA models, each with their own set of advantages and disadvantages. Some of these models are larger and slower, but may produce better results for datasets that are far outside their training distribution. Other models are smaller and faster, but their results may be less useful for images falling far outside their training distribution. Currently, the available models for captioning are:\n",
"- ViT-GPT2 : `'vitgpt2'` : a lightweight and fast model trained on COCO images. This model takes about 0.5s per image caption (on a CPU), but may provide less useful results for images that are very different from COCO-like images.\n",
"- BLIP-2 : `'blip2'` : a more heavyweight model. This model may provide more robust answers for images different than COCO images, but can take upwards of 10s per image caption.\n",
"- BLIP : `'blip'` : a middleweight model that provides a middle-way approach between ViT-GPT2 and BLIP-2.\n",
"\n",
"Available models for VQA are:\n",
"- Vilt-b32: `'vqa'` : a fairly lightweight model used for question answering.\n",
"- ViT-Age: `'age'` : a lightweight model used to classify the age of humans in a photo.\n",
"---> used for person age VQA\n",
"\n",
"By default, the captioning model used will be ViT-GPT2, if not specified otherwise.\n",
"\n",
"**Selecting GPU/CPU and batch sizes:**\n",
"
The captioning method in fastdup enables you to select either a GPU or CPU for computation, and decide your preferred batch size. By default, CPU computation is selected, and batch sizes are set to 8. For GPU's with high-RAM (40GB), a batch size of 256 will enable captioning in under 0.05 seconds per image."
]
},
{
"cell_type": "code",
"execution_count": null,
"metadata": {},
"outputs": [],
"source": [
"captions_df = fd.caption(model_name='automatic', device = 'cpu', batch_size=8)"
]
},
{
"cell_type": "code",
"execution_count": 10,
"metadata": {},
"outputs": [
{
"data": {
"text/html": [
"
\n",
"\n",
"
\n",
" \n",
" \n",
" | \n",
" filename | \n",
" caption | \n",
"
\n",
" \n",
" \n",
" \n",
" 230 | \n",
" coco_minitrain_25k/images/train2017/000000005811.jpg | \n",
" a red bus is driving down the street | \n",
"
\n",
" \n",
" 398 | \n",
" coco_minitrain_25k/images/train2017/000000009845.jpg | \n",
" a man is standing next to a bus | \n",
"
\n",
" \n",
" 803 | \n",
" coco_minitrain_25k/images/train2017/000000019167.jpg | \n",
" a bowl of oranges in a metal bowl | \n",
"
\n",
" \n",
" 493 | \n",
" coco_minitrain_25k/images/train2017/000000012315.jpg | \n",
" a cat is sitting on a toilet seat | \n",
"
\n",
" \n",
" 162 | \n",
" coco_minitrain_25k/images/train2017/000000004404.jpg | \n",
" a woman walking down a street holding an umbrella | \n",
"
\n",
" \n",
"
\n",
"
"
],
"text/plain": [
" filename caption\n",
"230 coco_minitrain_25k/images/train2017/000000005811.jpg a red bus is driving down the street \n",
"398 coco_minitrain_25k/images/train2017/000000009845.jpg a man is standing next to a bus \n",
"803 coco_minitrain_25k/images/train2017/000000019167.jpg a bowl of oranges in a metal bowl \n",
"493 coco_minitrain_25k/images/train2017/000000012315.jpg a cat is sitting on a toilet seat \n",
"162 coco_minitrain_25k/images/train2017/000000004404.jpg a woman walking down a street holding an umbrella "
]
},
"execution_count": 10,
"metadata": {},
"output_type": "execute_result"
}
],
"source": [
"captions_sample = captions_df.sample(n=5)\n",
"captions_sample.loc[:,['filename', 'caption']].head(5)"
]
},
{
"cell_type": "markdown",
"metadata": {},
"source": [
"# Visualizing the Dataset's Outlier Images With Their Captions\n",
"\n",
"Use fastdup's built-in galleries methods to visualize the captioned images.\n",
"Additionally, captions can always be generated for a gallery by setting the `label_col` argument to one of the available model names listed above."
]
},
{
"cell_type": "code",
"execution_count": 14,
"metadata": {},
"outputs": [
{
"name": "stderr",
"output_type": "stream",
"text": [
"100%|██████████| 10/10 [00:00<00:00, 23643.20it/s]"
]
},
{
"name": "stdout",
"output_type": "stream",
"text": [
"Stored outliers visual view in ./outliers.html\n"
]
},
{
"name": "stderr",
"output_type": "stream",
"text": [
"\n"
]
},
{
"data": {
"text/plain": [
"0"
]
},
"execution_count": 14,
"metadata": {},
"output_type": "execute_result"
}
],
"source": [
"import pandas as pd\n",
"captions_to_show = captions_df.sample(20)\n",
"visualization_df = pd.DataFrame({'from':captions_to_show['filename'],'to':captions_to_show['filename'], 'label':captions_to_show['caption'], 'distance':0*len(captions_to_show),})\n",
"fastdup.create_outliers_gallery(visualization_df, save_path='.', num_images=10)"
]
},
{
"cell_type": "code",
"execution_count": 15,
"metadata": {},
"outputs": [
{
"data": {
"text/html": [
" \n",
" \n",
" \n",
" \n",
" \n",
" \n",
" \n",
" \n",
" \n",
" \n",
" \n",
" \n",
" \n",
" \n",
" \n",
" \n",
" Outliers Report\n",
" \n",
" \n",
"\n",
"\n",
"\n",
" \n",
" \n",
" \n",
" \n",
" \n",
"
\n",
"
\n",
"
Outliers Report
Showing image outliers, one per row
\n",
"
\n",
"
\n",
"
\n",
" \n",
" \n",
" \n",
"
\n",
"
\n",
"

\n",
"
\n",
"
\n",
"
\n",
"
\n",
" \n",
" \n",
" Info | \n",
"
\n",
"\n",
" Distance | \n",
" 0 | \n",
"
\n",
"\n",
" Path | \n",
" coco_minitrain_25k/images/train2017/000000001737.jpg | \n",
"
\n",
"\n",
" label | \n",
" two polar bears are sitting on rocks in the wilderness | \n",
"
\n",
" \n",
"
\n",
"
\n",
"
\n",
"
\n",
"
\n",
"
\n",
"

\n",
"
\n",
"
\n",
"
\n",
"
\n",
" \n",
" \n",
" Info | \n",
"
\n",
"\n",
" Distance | \n",
" 0 | \n",
"
\n",
"\n",
" Path | \n",
" coco_minitrain_25k/images/train2017/000000003538.jpg | \n",
"
\n",
"\n",
" label | \n",
" a person on skis is going down a hill | \n",
"
\n",
" \n",
"
\n",
"
\n",
"
\n",
"
\n",
"
\n",
"
\n",
"

\n",
"
\n",
"
\n",
"
\n",
"
\n",
" \n",
" \n",
" Info | \n",
"
\n",
"\n",
" Distance | \n",
" 0 | \n",
"
\n",
"\n",
" Path | \n",
" coco_minitrain_25k/images/train2017/000000011305.jpg | \n",
"
\n",
"\n",
" label | \n",
" a living room with a couch, a table and a window | \n",
"
\n",
" \n",
"
\n",
"
\n",
"
\n",
"
\n",
"
\n",
"
\n",
"

\n",
"
\n",
"
\n",
"
\n",
"
\n",
" \n",
" \n",
" Info | \n",
"
\n",
"\n",
" Distance | \n",
" 0 | \n",
"
\n",
"\n",
" Path | \n",
" coco_minitrain_25k/images/train2017/000000004820.jpg | \n",
"
\n",
"\n",
" label | \n",
" a cat laying on a bed with a blanket | \n",
"
\n",
" \n",
"
\n",
"
\n",
"
\n",
"
\n",
"
\n",
"
\n",
"

\n",
"
\n",
"
\n",
"
\n",
"
\n",
" \n",
" \n",
" Info | \n",
"
\n",
"\n",
" Distance | \n",
" 0 | \n",
"
\n",
"\n",
" Path | \n",
" coco_minitrain_25k/images/train2017/000000020438.jpg | \n",
"
\n",
"\n",
" label | \n",
" a dog laying on a bed with a blanket | \n",
"
\n",
" \n",
"
\n",
"
\n",
"
\n",
"
\n",
"
\n",
"
\n",
"

\n",
"
\n",
"
\n",
"
\n",
"
\n",
" \n",
" \n",
" Info | \n",
"
\n",
"\n",
" Distance | \n",
" 0 | \n",
"
\n",
"\n",
" Path | \n",
" coco_minitrain_25k/images/train2017/000000010489.jpg | \n",
"
\n",
"\n",
" label | \n",
" a bathroom with a toilet, sink, and tub | \n",
"
\n",
" \n",
"
\n",
"
\n",
"
\n",
"
\n",
"
\n",
"
\n",
"

\n",
"
\n",
"
\n",
"
\n",
"
\n",
" \n",
" \n",
" Info | \n",
"
\n",
"\n",
" Distance | \n",
" 0 | \n",
"
\n",
"\n",
" Path | \n",
" coco_minitrain_25k/images/train2017/000000016716.jpg | \n",
"
\n",
"\n",
" label | \n",
" two zebras standing in a field of grass | \n",
"
\n",
" \n",
"
\n",
"
\n",
"
\n",
"
\n",
"
\n",
"
\n",
"

\n",
"
\n",
"
\n",
"
\n",
"
\n",
" \n",
" \n",
" Info | \n",
"
\n",
"\n",
" Distance | \n",
" 0 | \n",
"
\n",
"\n",
" Path | \n",
" coco_minitrain_25k/images/train2017/000000023194.jpg | \n",
"
\n",
"\n",
" label | \n",
" a horse drawn carriage on a street | \n",
"
\n",
" \n",
"
\n",
"
\n",
"
\n",
"
\n",
"
\n",
"
\n",
"

\n",
"
\n",
"
\n",
"
\n",
"
\n",
" \n",
" \n",
" Info | \n",
"
\n",
"\n",
" Distance | \n",
" 0 | \n",
"
\n",
"\n",
" Path | \n",
" coco_minitrain_25k/images/train2017/000000010130.jpg | \n",
"
\n",
"\n",
" label | \n",
" a woman is looking at her cell phone | \n",
"
\n",
" \n",
"
\n",
"
\n",
"
\n",
"
\n",
"
\n",
"
\n",
"

\n",
"
\n",
"
\n",
"
\n",
"
\n",
" \n",
" \n",
" Info | \n",
"
\n",
"\n",
" Distance | \n",
" 0 | \n",
"
\n",
"\n",
" Path | \n",
" coco_minitrain_25k/images/train2017/000000018633.jpg | \n",
"
\n",
"\n",
" label | \n",
" two dogs are standing outside of a door | \n",
"
\n",
" \n",
"
\n",
"
\n",
"
\n",
"
\n",
" \n",
"
\n",
" \n",
" \n",
" \n",
" \n",
" "
],
"text/plain": [
""
]
},
"execution_count": 15,
"metadata": {},
"output_type": "execute_result"
}
],
"source": [
"from IPython.display import HTML\n",
"HTML('outliers.html')"
]
},
{
"cell_type": "markdown",
"metadata": {},
"source": [
"# Visual Question Answering"
]
},
{
"cell_type": "markdown",
"metadata": {},
"source": [
"Visual question answering in fastdup allows you to use open-ended questions to learn more about the images in your dataset. These can be questions such as \"is this photo taken indoors or outdoors?\", \"is there a dog in the photo?\", \"is this a photo of an animal or an object?\", or any other questions that come to mind. The output from these queries can, in turn, be used for image retrieval, to aid the visually impaired, and many other interesting use cases."
]
},
{
"cell_type": "code",
"execution_count": null,
"metadata": {},
"outputs": [],
"source": [
"vqa_df = fd.caption(model_name='vqa', vqa_prompt='is this photo taken indoors or outdoors?')"
]
},
{
"cell_type": "code",
"execution_count": 17,
"metadata": {},
"outputs": [
{
"data": {
"text/html": [
"\n",
"\n",
"
\n",
" \n",
" \n",
" | \n",
" filename | \n",
" caption | \n",
"
\n",
" \n",
" \n",
" \n",
" 702 | \n",
" coco_minitrain_25k/images/train2017/000000017004.jpg | \n",
" indoors | \n",
"
\n",
" \n",
" 768 | \n",
" coco_minitrain_25k/images/train2017/000000018464.jpg | \n",
" outdoors | \n",
"
\n",
" \n",
" 338 | \n",
" coco_minitrain_25k/images/train2017/000000008458.jpg | \n",
" outdoors | \n",
"
\n",
" \n",
" 164 | \n",
" coco_minitrain_25k/images/train2017/000000004502.jpg | \n",
" outdoors | \n",
"
\n",
" \n",
" 587 | \n",
" coco_minitrain_25k/images/train2017/000000014271.jpg | \n",
" outdoors | \n",
"
\n",
" \n",
"
\n",
"
"
],
"text/plain": [
" filename caption\n",
"702 coco_minitrain_25k/images/train2017/000000017004.jpg indoors\n",
"768 coco_minitrain_25k/images/train2017/000000018464.jpg outdoors\n",
"338 coco_minitrain_25k/images/train2017/000000008458.jpg outdoors\n",
"164 coco_minitrain_25k/images/train2017/000000004502.jpg outdoors\n",
"587 coco_minitrain_25k/images/train2017/000000014271.jpg outdoors"
]
},
"execution_count": 17,
"metadata": {},
"output_type": "execute_result"
}
],
"source": [
"vqa_sample = vqa_df.sample(n=5)\n",
"vqa_sample.loc[:,['filename', 'caption']].head(5)"
]
},
{
"cell_type": "markdown",
"metadata": {},
"source": [
"# Visualize the Results of VQA on The Dataset's Outliers\n",
"\n",
"Once again, we will use fastdup's built-in galleries methods to visualize the results of our VQA prompts."
]
},
{
"cell_type": "code",
"execution_count": 18,
"metadata": {},
"outputs": [
{
"name": "stderr",
"output_type": "stream",
"text": [
"100%|██████████| 10/10 [00:00<00:00, 21597.86it/s]"
]
},
{
"name": "stdout",
"output_type": "stream",
"text": [
"Stored outliers visual view in ./outliers.html\n"
]
},
{
"name": "stderr",
"output_type": "stream",
"text": [
"\n"
]
},
{
"data": {
"text/plain": [
"0"
]
},
"execution_count": 18,
"metadata": {},
"output_type": "execute_result"
}
],
"source": [
"vqa_to_show = vqa_df.sample(20)\n",
"vis_vqa_df = pd.DataFrame({'from':vqa_to_show['filename'],'to':vqa_to_show['filename'], 'label':vqa_to_show['caption'], 'distance':0*len(vqa_to_show),})\n",
"fastdup.create_outliers_gallery(vis_vqa_df, save_path='.', num_images=10)"
]
},
{
"cell_type": "code",
"execution_count": 19,
"metadata": {},
"outputs": [
{
"data": {
"text/html": [
" \n",
" \n",
" \n",
" \n",
" \n",
" \n",
" \n",
" \n",
" \n",
" \n",
" \n",
" \n",
" \n",
" \n",
" \n",
" \n",
" Outliers Report\n",
" \n",
" \n",
"\n",
"\n",
"\n",
" \n",
" \n",
" \n",
" \n",
" \n",
"
\n",
"
\n",
"
Outliers Report
Showing image outliers, one per row
\n",
"
\n",
"
\n",
"
\n",
" \n",
" \n",
" \n",
"
\n",
"
\n",
"

\n",
"
\n",
"
\n",
"
\n",
"
\n",
" \n",
" \n",
" Info | \n",
"
\n",
"\n",
" Distance | \n",
" 0 | \n",
"
\n",
"\n",
" Path | \n",
" coco_minitrain_25k/images/train2017/000000012475.jpg | \n",
"
\n",
"\n",
" label | \n",
" outdoors | \n",
"
\n",
" \n",
"
\n",
"
\n",
"
\n",
"
\n",
"
\n",
"
\n",
"

\n",
"
\n",
"
\n",
"
\n",
"
\n",
" \n",
" \n",
" Info | \n",
"
\n",
"\n",
" Distance | \n",
" 0 | \n",
"
\n",
"\n",
" Path | \n",
" coco_minitrain_25k/images/train2017/000000005344.jpg | \n",
"
\n",
"\n",
" label | \n",
" outdoors | \n",
"
\n",
" \n",
"
\n",
"
\n",
"
\n",
"
\n",
"
\n",
"
\n",
"

\n",
"
\n",
"
\n",
"
\n",
"
\n",
" \n",
" \n",
" Info | \n",
"
\n",
"\n",
" Distance | \n",
" 0 | \n",
"
\n",
"\n",
" Path | \n",
" coco_minitrain_25k/images/train2017/000000010324.jpg | \n",
"
\n",
"\n",
" label | \n",
" indoors | \n",
"
\n",
" \n",
"
\n",
"
\n",
"
\n",
"
\n",
"
\n",
"
\n",
"

\n",
"
\n",
"
\n",
"
\n",
"
\n",
" \n",
" \n",
" Info | \n",
"
\n",
"\n",
" Distance | \n",
" 0 | \n",
"
\n",
"\n",
" Path | \n",
" coco_minitrain_25k/images/train2017/000000005139.jpg | \n",
"
\n",
"\n",
" label | \n",
" outdoors | \n",
"
\n",
" \n",
"
\n",
"
\n",
"
\n",
"
\n",
"
\n",
"
\n",
"

\n",
"
\n",
"
\n",
"
\n",
"
\n",
" \n",
" \n",
" Info | \n",
"
\n",
"\n",
" Distance | \n",
" 0 | \n",
"
\n",
"\n",
" Path | \n",
" coco_minitrain_25k/images/train2017/000000002614.jpg | \n",
"
\n",
"\n",
" label | \n",
" outdoors | \n",
"
\n",
" \n",
"
\n",
"
\n",
"
\n",
"
\n",
"
\n",
"
\n",
"

\n",
"
\n",
"
\n",
"
\n",
"
\n",
" \n",
" \n",
" Info | \n",
"
\n",
"\n",
" Distance | \n",
" 0 | \n",
"
\n",
"\n",
" Path | \n",
" coco_minitrain_25k/images/train2017/000000005540.jpg | \n",
"
\n",
"\n",
" label | \n",
" indoors | \n",
"
\n",
" \n",
"
\n",
"
\n",
"
\n",
"
\n",
"
\n",
"
\n",
"

\n",
"
\n",
"
\n",
"
\n",
"
\n",
" \n",
" \n",
" Info | \n",
"
\n",
"\n",
" Distance | \n",
" 0 | \n",
"
\n",
"\n",
" Path | \n",
" coco_minitrain_25k/images/train2017/000000005107.jpg | \n",
"
\n",
"\n",
" label | \n",
" outdoors | \n",
"
\n",
" \n",
"
\n",
"
\n",
"
\n",
"
\n",
"
\n",
"
\n",
"

\n",
"
\n",
"
\n",
"
\n",
"
\n",
" \n",
" \n",
" Info | \n",
"
\n",
"\n",
" Distance | \n",
" 0 | \n",
"
\n",
"\n",
" Path | \n",
" coco_minitrain_25k/images/train2017/000000023811.jpg | \n",
"
\n",
"\n",
" label | \n",
" outdoors | \n",
"
\n",
" \n",
"
\n",
"
\n",
"
\n",
"
\n",
"
\n",
"
\n",
"

\n",
"
\n",
"
\n",
"
\n",
"
\n",
" \n",
" \n",
" Info | \n",
"
\n",
"\n",
" Distance | \n",
" 0 | \n",
"
\n",
"\n",
" Path | \n",
" coco_minitrain_25k/images/train2017/000000001625.jpg | \n",
"
\n",
"\n",
" label | \n",
" outdoors | \n",
"
\n",
" \n",
"
\n",
"
\n",
"
\n",
"
\n",
"
\n",
"
\n",
"

\n",
"
\n",
"
\n",
"
\n",
"
\n",
" \n",
" \n",
" Info | \n",
"
\n",
"\n",
" Distance | \n",
" 0 | \n",
"
\n",
"\n",
" Path | \n",
" coco_minitrain_25k/images/train2017/000000012754.jpg | \n",
"
\n",
"\n",
" label | \n",
" indoors | \n",
"
\n",
" \n",
"
\n",
"
\n",
"
\n",
"
\n",
" \n",
"
\n",
" \n",
" \n",
" \n",
" \n",
" "
],
"text/plain": [
""
]
},
"execution_count": 19,
"metadata": {},
"output_type": "execute_result"
}
],
"source": [
"from IPython.display import HTML\n",
"HTML('outliers.html')"
]
},
{
"cell_type": "markdown",
"metadata": {},
"source": [
"## Wrap Up\n",
"\n",
"That's a wrap! In this notebook we showed how you load dataset from Kaggle and analyze it using fastdup. You can use similar methods to run on other similar datasets on [Roboflow Universe](https://universe.roboflow.com/).\n",
"\n",
"Try it out and let us know what issues you find.\n",
"\n",
"Next, feel free to check out other tutorials -\n",
"\n",
"+ ⚡ [**Quickstart**](https://nbviewer.org/github/visual-layer/fastdup/blob/main/examples/quick-dataset-analysis.ipynb): Learn how to install fastdup, load a dataset and analyze it for potential issues such as duplicates/near-duplicates, broken images, outliers, dark/bright/blurry images, and view visually similar image clusters. If you're new, start here!\n",
"+ 🧹 [**Clean Image Folder**](https://nbviewer.org/github/visual-layer/fastdup/blob/main/examples/cleaning-image-dataset.ipynb): Learn how to analyze and clean a folder of images from potential issues and export a list of problematic files for further action. If you have an unorganized folder of images, this is a good place to start.\n",
"+ 🖼 [**Analyze Image Classification Dataset**](https://nbviewer.org/github/visual-layer/fastdup/blob/main/examples/analyzing-image-classification-dataset.ipynb): Learn how to load a labeled image classification dataset and analyze for potential issues. If you have labeled ImageNet-style folder structure, have a go!\n",
"+ 🎁 [**Analyze Object Detection Dataset**](https://nbviewer.org/github/visual-layer/fastdup/blob/main/examples/analyzing-object-detection-dataset.ipynb): Learn how to load bounding box annotations for object detection and analyze for potential issues. If you have a COCO-style labeled object detection dataset, give this example a try. "
]
},
{
"cell_type": "markdown",
"metadata": {},
"source": [
"\n",
"## VL Profiler - A faster and easier way to diagnose and visualize dataset issues\n",
"\n",
"If you prefer a no-code platform to inspect and visualize your dataset, [**try our free cloud product VL Profiler**](https://app.visual-layer.com) - VL Profiler is our first no-code commercial product that lets you visualize and inspect your dataset in your browser. \n",
"\n",
"VL Profiler is free to get started. Upload up to 1,000,000 images for analysis at zero cost!\n",
"\n",
"[Sign up](https://app.visual-layer.com) now.\n",
"\n",
"[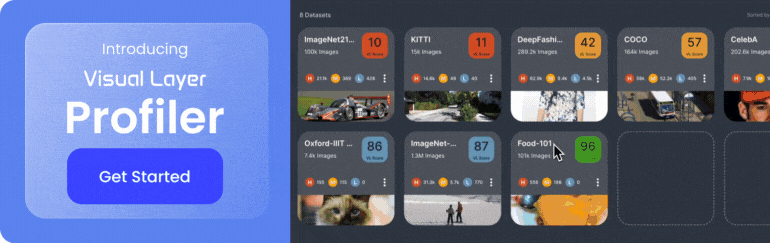](https://app.visual-layer.com)\n",
"\n",
"As usual, feedback is welcome! Questions? Drop by our [Slack channel](https://visualdatabase.slack.com/join/shared_invite/zt-19jaydbjn-lNDEDkgvSI1QwbTXSY6dlA#/shared-invite/email) or open an issue on [GitHub](https://github.com/visual-layer/fastdup/issues)."
]
},
{
"cell_type": "markdown",
"metadata": {},
"source": [
" \n",
"
\n",
" GitHub •\n",
" Join Slack Community •\n",
" Discussion Forum \n",
"\n",
"\n",
" \n",
" Blog •\n",
" Documentation •\n",
" About Us \n",
"\n",
"\n",
" \n",
" LinkedIn •\n",
" Twitter \n",
""
]
}
],
"metadata": {
"kernelspec": {
"display_name": "Python 3",
"language": "python",
"name": "python3"
},
"language_info": {
"codemirror_mode": {
"name": "ipython",
"version": 3
},
"file_extension": ".py",
"mimetype": "text/x-python",
"name": "python",
"nbconvert_exporter": "python",
"pygments_lexer": "ipython3",
"version": "3.9.6"
},
"orig_nbformat": 4
},
"nbformat": 4,
"nbformat_minor": 2
}