15.5 Logistic regression with glm(family = "binomial"
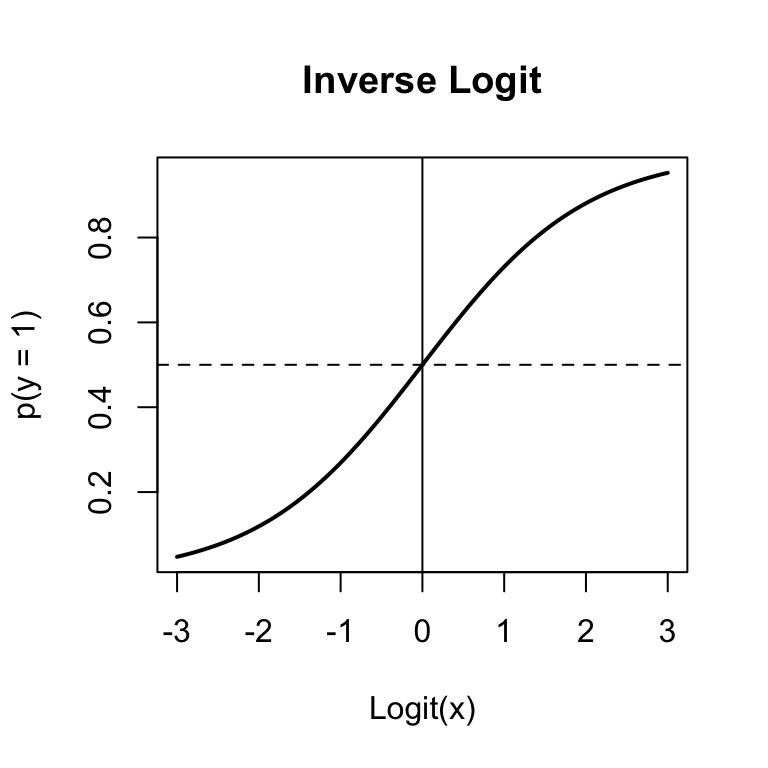
Figure 15.3: The inverse logit function used in binary logistic regression to convert logits to probabilities.
The most common non-normal regression analysis is logistic regression, where your dependent variable is just 0s and 1. To do a logistic regression analysis with glm()
, use the family = binomial
argument.
Let’s run a logistic regression on the diamonds dataset. First, I’ll create a binary variable called value.g190
indicating whether the value of a diamond is greater than 190 or not. Then, I’ll conduct a logistic regression with our new binary variable as the dependent variable. We’ll set family = "binomial"
to tell glm()
that the dependent variable is binary.
# Create a binary variable indicating whether or not
# a diamond's value is greater than 190
diamonds$value.g190 <- diamonds$value > 190
# Conduct a logistic regression on the new binary variable
diamond.glm <- glm(formula = value.g190 ~ weight + clarity + color,
data = diamonds,
family = binomial)
Here are the resulting coefficients:
# Print coefficients from logistic regression
summary(diamond.glm)$coefficients
## Estimate Std. Error z value Pr(>|z|)
## (Intercept) -18.8009 3.4634 -5.428 5.686e-08
## weight 1.1251 0.1968 5.716 1.088e-08
## clarity 9.2910 1.9629 4.733 2.209e-06
## color -0.3836 0.2481 -1.547 1.220e-01
Just like with regular regression with lm()
, we can get the fitted values from the model and put them back into our dataset to see how well the model fit the data:
# Add logistic fitted values back to dataframe as
# new column pred.g190
diamonds$pred.g190 <- diamond.glm$fitted.values
# Look at the first few rows (of the named columns)
head(diamonds[c("weight", "clarity", "color", "value", "pred.g190")])
## weight clarity color value pred.g190
## 1 9.35 0.88 4 182.5 0.16252
## 2 11.10 1.05 5 191.2 0.82130
## 3 8.65 0.85 6 175.7 0.03008
## 4 10.43 1.15 5 195.2 0.84559
## 5 10.62 0.92 5 181.6 0.44455
## 6 12.35 0.44 4 182.9 0.08688
Looking at the first few observations, it looks like the probabilities match the data pretty well. For example, the first diamond with a value of 182.5 had a fitted probability of just 0.16 of being valued greater than 190. In contrast, the second diamond, which had a value of 191.2 had a much higher fitted probability of 0.82.
Just like we did with regular regression, you can use the predict()
function along with the results of a glm()
object to predict new data. Let’s use the diamond.glm
object to predict the probability that the new diamonds will have a value greater than 190:
# Predict the 'probability' that the 3 new diamonds
# will have a value greater than 190
predict(object = diamond.glm,
newdata = diamonds.new)
## 1 2 3
## 4.8605 -3.5265 -0.3898
What the heck, these don’t look like probabilities! True, they’re not. They are logit-transformed probabilities. To turn them back into probabilities, we need to invert them by applying the inverse logit function:
# Get logit predictions of new diamonds
logit.predictions <- predict(object = diamond.glm,
newdata = diamonds.new
)
# Apply inverse logit to transform to probabilities
# (See Equation in the margin)
prob.predictions <- 1 / (1 + exp(-logit.predictions))
# Print final predictions!
prob.predictions
## 1 2 3
## 0.99231 0.02857 0.40376
So, the model predicts that the probability that the three new diamonds will be valued over 190 is 99.23%, 2.86%, and 40.38% respectively.
15.5.1 Adding a regression line to a plot
You can easily add a regression line to a scatterplot. To do this, just put the regression object you created with as the main argument to . For example, the following code will create the scatterplot on the right (Figure~\(\ref{fig:scatterreg}\)) showing the relationship between a diamond’s weight and its value including a red regression line:
# Scatterplot of diamond weight and value
plot(x = diamonds$weight,
y = diamonds$value,
xlab = "Weight",
ylab = "Value",
main = "Adding a regression line with abline()"
)
# Calculate regression model
diamonds.lm <- lm(formula = value ~ weight,
data = diamonds)
# Add regression line
abline(diamonds.lm,
col = "red", lwd = 2)
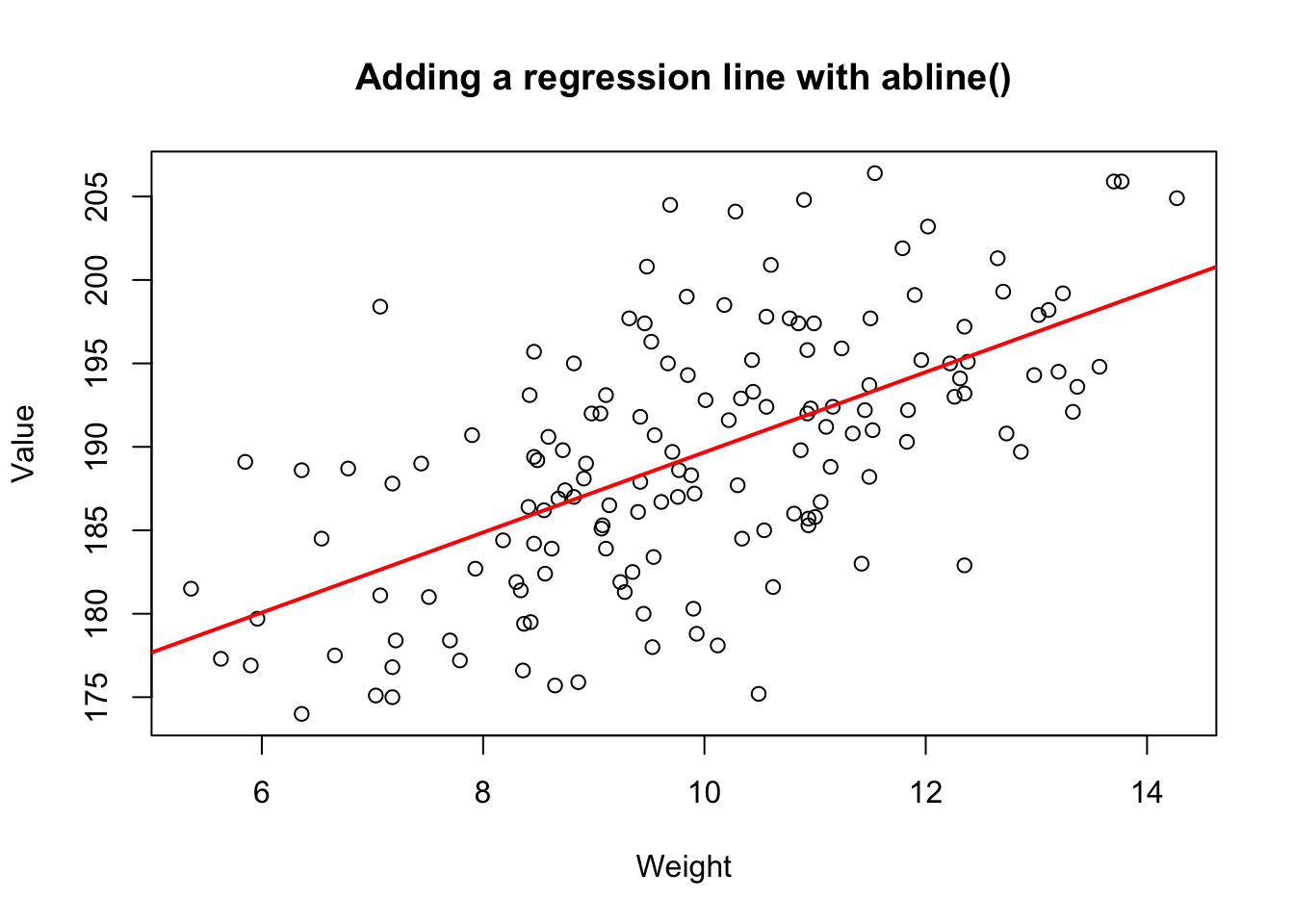
Figure 15.4: Adding a regression line to a scatterplot using abline()
15.5.2 Transforming skewed variables prior to standard regression
# The distribution of movie revenus is highly
# skewed.
hist(movies$revenue.all,
main = "Movie revenue\nBefore log-transformation")
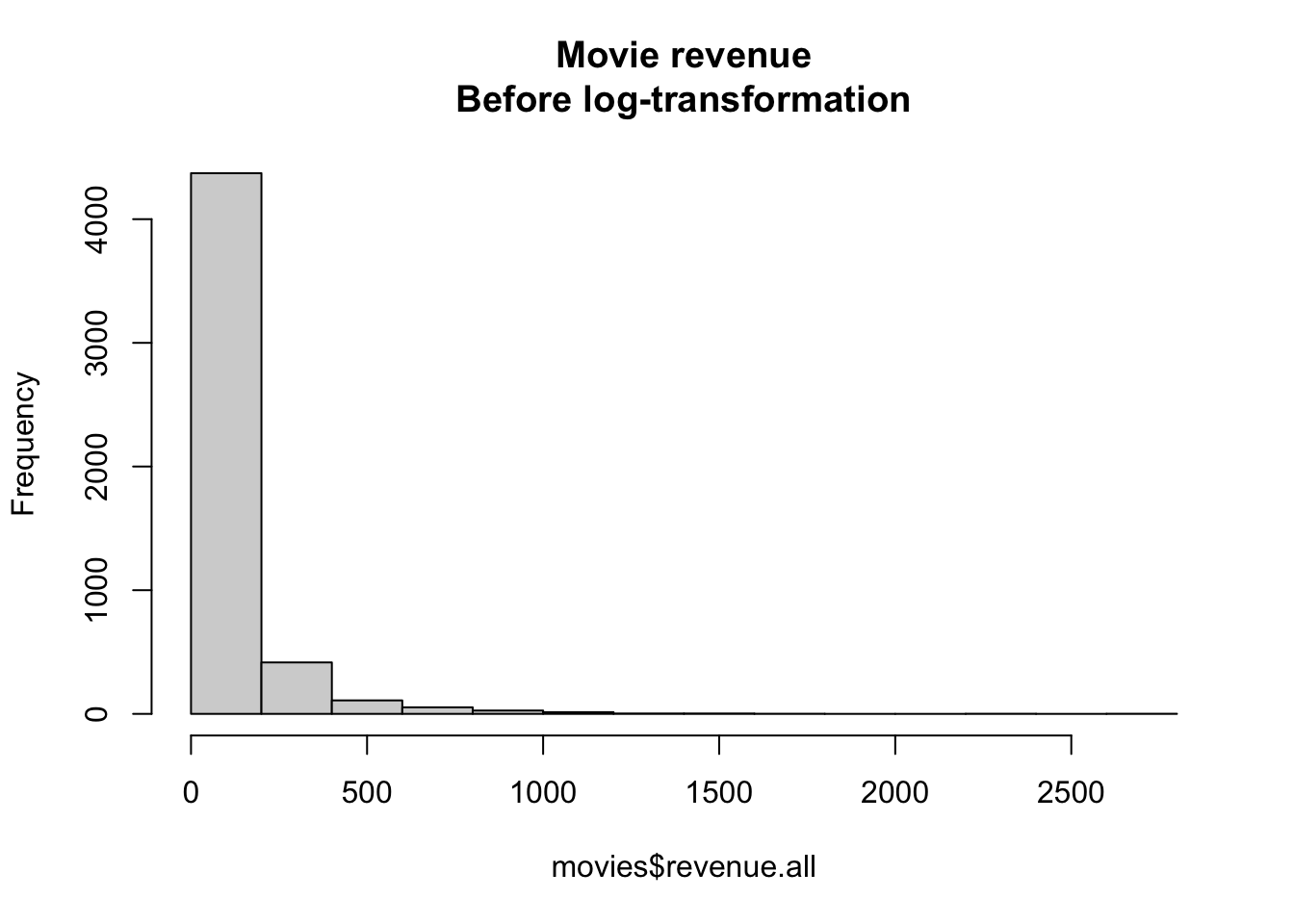
Figure 15.5: Distribution of movie revenues without a log-transformation
If you have a highly skewed variable that you want to include in a regression analysis, you can do one of two things. Option 1 is to use the general linear model glm()
with an appropriate family (like family = "gamma"
). Option 2 is to do a standard regression analysis with lm()
, but before doing so, transforming the variable into something less skewed. For highly skewed data, the most common transformation is a log-transformation.
For example, look at the distribution of movie revenues in the movies dataset in the margin Figure 15.5:
As you can see, these data don’t look Normally distributed at all. There are a few movies (like Avatar) that just an obscene amount of money, and many movies that made much less. If we want to conduct a standard regression analysis on these data, we need to create a new log-transformed version of the variable. In the following code, I’ll create a new variable called revenue.all.log
defined as the logarithm of revenue.all
# Create a new log-transformed version of movie revenue
movies$revenue.all.log <- log(movies$revenue.all)
In Figure 15.6 you can see a histogram of the new log-transformed variable. It’s still skewed, but not nearly as badly as before, so I would be feel much better using this variable in a standard regression analysis with lm()
.
# Distribution of log-transformed
# revenue is much less skewed
hist(movies$revenue.all.log,
main = "Log-transformed Movie revenue")
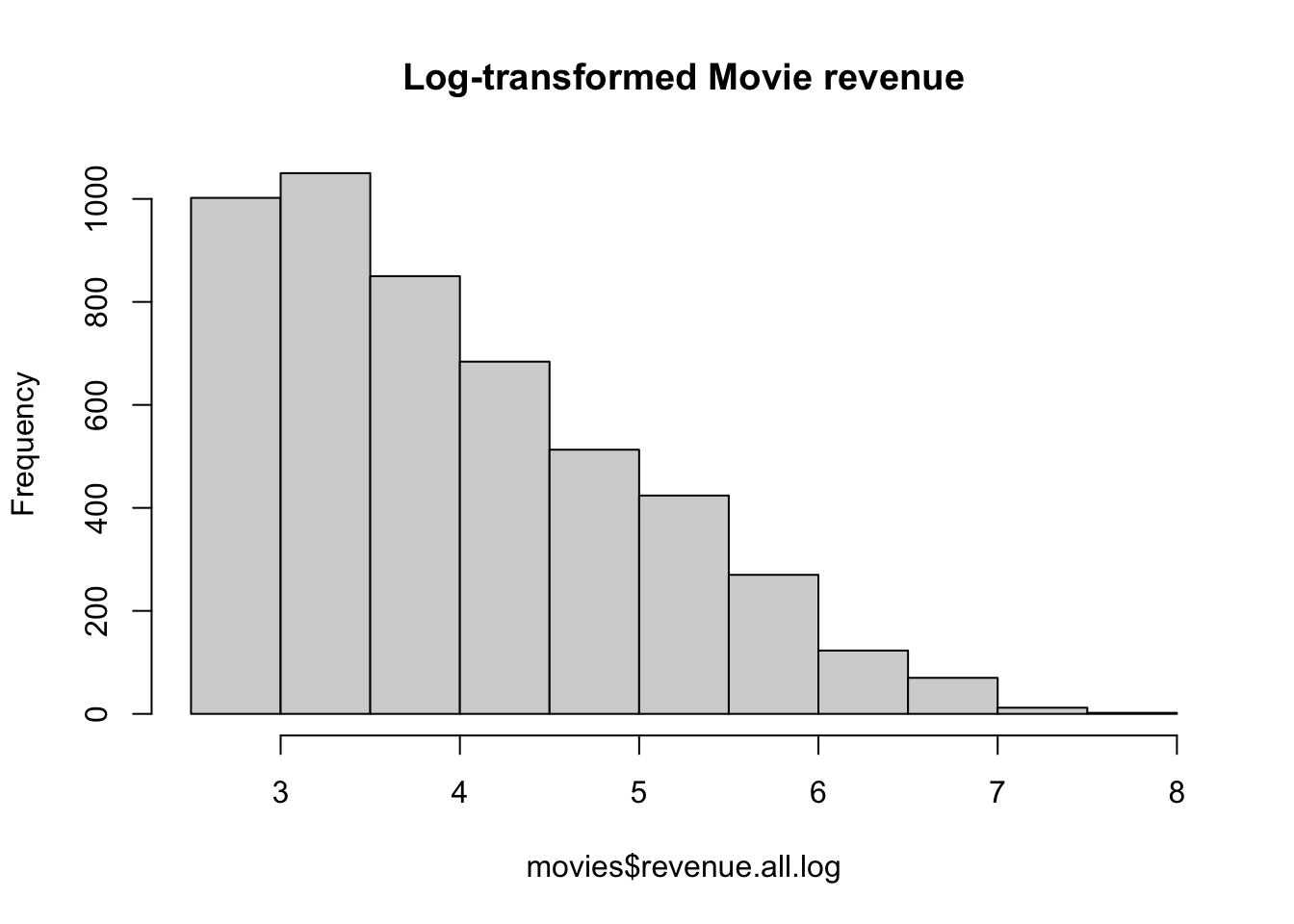
Figure 15.6: Distribution of log-transformed movie revenues. It’s still skewed, but not nearly as badly as before.