Note
Go to the end to download the full example code. or to run this example in your browser via JupyterLite or Binder
Plot classification probability#
Plot the classification probability for different classifiers. We use a 3 class dataset, and we classify it with a Support Vector classifier, L1 and L2 penalized logistic regression (multinomial multiclass), a One-Vs-Rest version with logistic regression, and Gaussian process classification.
Linear SVC is not a probabilistic classifier by default but it has a built-in
calibration option enabled in this example (probability=True
).
The logistic regression with One-Vs-Rest is not a multiclass classifier out of the box. As a result it has more trouble in separating class 2 and 3 than the other estimators.
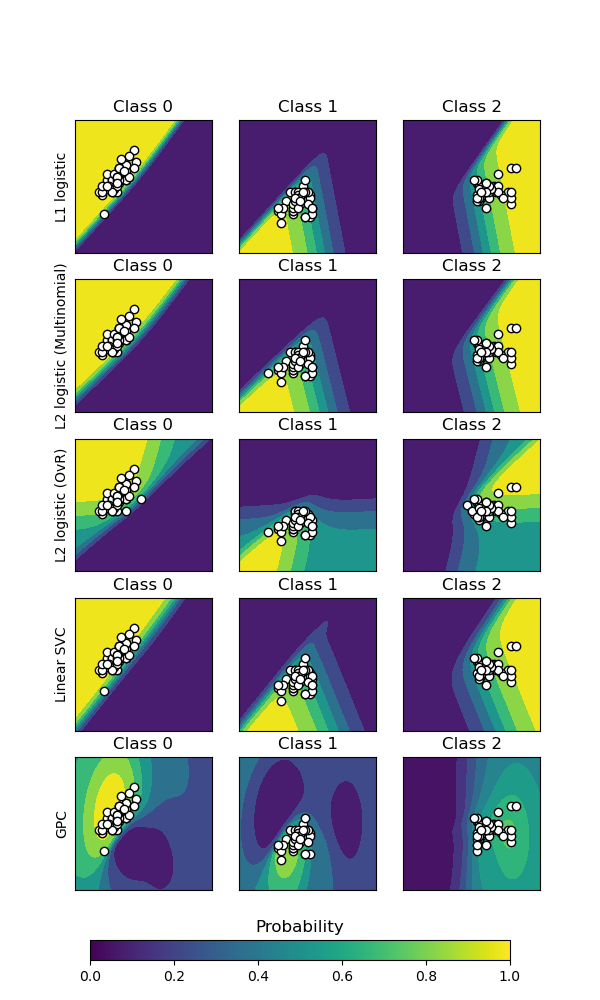
Accuracy (train) for L1 logistic: 83.3%
Accuracy (train) for L2 logistic (Multinomial): 82.7%
Accuracy (train) for L2 logistic (OvR): 79.3%
Accuracy (train) for Linear SVC: 82.0%
Accuracy (train) for GPC: 82.7%
# Authors: The scikit-learn developers
# SPDX-License-Identifier: BSD-3-Clause
import matplotlib.pyplot as plt
import numpy as np
from matplotlib import cm
from sklearn import datasets
from sklearn.gaussian_process import GaussianProcessClassifier
from sklearn.gaussian_process.kernels import RBF
from sklearn.inspection import DecisionBoundaryDisplay
from sklearn.linear_model import LogisticRegression
from sklearn.metrics import accuracy_score
from sklearn.multiclass import OneVsRestClassifier
from sklearn.svm import SVC
iris = datasets.load_iris()
X = iris.data[:, 0:2] # we only take the first two features for visualization
y = iris.target
n_features = X.shape[1]
C = 10
kernel = 1.0 * RBF([1.0, 1.0]) # for GPC
# Create different classifiers.
classifiers = {
"L1 logistic": LogisticRegression(C=C, penalty="l1", solver="saga", max_iter=10000),
"L2 logistic (Multinomial)": LogisticRegression(
C=C, penalty="l2", solver="saga", max_iter=10000
),
"L2 logistic (OvR)": OneVsRestClassifier(
LogisticRegression(C=C, penalty="l2", solver="saga", max_iter=10000)
),
"Linear SVC": SVC(kernel="linear", C=C, probability=True, random_state=0),
"GPC": GaussianProcessClassifier(kernel),
}
n_classifiers = len(classifiers)
fig, axes = plt.subplots(
nrows=n_classifiers,
ncols=len(iris.target_names),
figsize=(3 * 2, n_classifiers * 2),
)
for classifier_idx, (name, classifier) in enumerate(classifiers.items()):
y_pred = classifier.fit(X, y).predict(X)
accuracy = accuracy_score(y, y_pred)
print(f"Accuracy (train) for {name}: {accuracy:0.1%}")
for label in np.unique(y):
# plot the probability estimate provided by the classifier
disp = DecisionBoundaryDisplay.from_estimator(
classifier,
X,
response_method="predict_proba",
class_of_interest=label,
ax=axes[classifier_idx, label],
vmin=0,
vmax=1,
)
axes[classifier_idx, label].set_title(f"Class {label}")
# plot data predicted to belong to given class
mask_y_pred = y_pred == label
axes[classifier_idx, label].scatter(
X[mask_y_pred, 0], X[mask_y_pred, 1], marker="o", c="w", edgecolor="k"
)
axes[classifier_idx, label].set(xticks=(), yticks=())
axes[classifier_idx, 0].set_ylabel(name)
ax = plt.axes([0.15, 0.04, 0.7, 0.02])
plt.title("Probability")
_ = plt.colorbar(
cm.ScalarMappable(norm=None, cmap="viridis"), cax=ax, orientation="horizontal"
)
plt.show()
Total running time of the script: (0 minutes 1.332 seconds)
Related examples
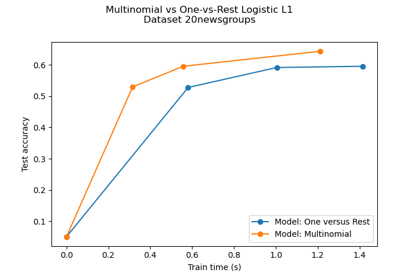
Multiclass sparse logistic regression on 20newgroups
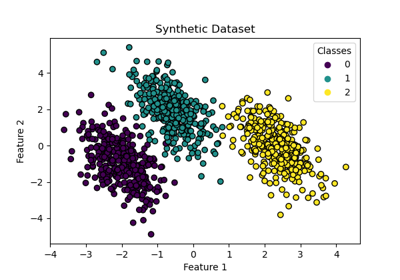
Decision Boundaries of Multinomial and One-vs-Rest Logistic Regression
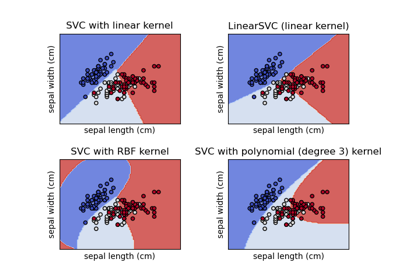
Plot different SVM classifiers in the iris dataset