Note
Go to the end to download the full example code.
Retieving wind drift factor from trajectory
from datetime import datetime, timedelta
import numpy as np
import matplotlib.pyplot as plt
import cmocean
from opendrift.models.oceandrift import OceanDrift
from opendrift.models.physics_methods import wind_drift_factor_from_trajectory, distance_between_trajectories, skillscore_liu_weissberg
A very simple drift model is: current + wind_drift_factor*wind where wind_drift_factor for surface drift is typically 0.033 if Stokes drift included, and 0.02 in addition to Stokes drift. This example illustrates how a best-fit wind_drift_factor can be calculated from an observed trajectory, using two different methods.
First we simulate a synthetic drifter trajectory
ot = OceanDrift(loglevel=50)
ot.add_readers_from_list([ot.test_data_folder() +
'16Nov2015_NorKyst_z_surface/norkyst800_subset_16Nov2015.nc',
ot.test_data_folder() + '16Nov2015_NorKyst_z_surface/arome_subset_16Nov2015.nc'], lazy=False)
/opt/conda/envs/opendrift/lib/python3.11/site-packages/pyproj/crs/crs.py:1286: UserWarning: You will likely lose important projection information when converting to a PROJ string from another format. See: https://proj.org/faq.html#what-is-the-best-format-for-describing-coordinate-reference-systems
proj = self._crs.to_proj4(version=version)
/opt/conda/envs/opendrift/lib/python3.11/site-packages/pyproj/crs/crs.py:1286: UserWarning: You will likely lose important projection information when converting to a PROJ string from another format. See: https://proj.org/faq.html#what-is-the-best-format-for-describing-coordinate-reference-systems
proj = self._crs.to_proj4(version=version)
Adding some horizontal diffusivity as “noise”
ot.set_config('drift:horizontal_diffusivity', 10)
Using a wind_drift_factor of 0.33 i.e. drift is current + 3.3% of wind speed
ot.seed_elements(lon=4, lat=60, number=1, time=ot.env.readers[list(ot.env.readers)[0]].start_time,
wind_drift_factor=0.033)
ot.run(duration=timedelta(hours=12), time_step=600)
Secondly, calculating the wind_drift_factor which reproduces the “observed” trajectory with minimal difference
drifter_lons = ot.history['lon'][0]
drifter_lats = ot.history['lat'][0]
drifter_times = ot.get_time_array()[0]
drifter={'lon': drifter_lons, 'lat': drifter_lats,
'time': drifter_times, 'linewidth': 2, 'color': 'b', 'label': 'Synthetic drifter'}
o = OceanDrift(loglevel=50)
o.add_readers_from_list([o.test_data_folder() +
'16Nov2015_NorKyst_z_surface/norkyst800_subset_16Nov2015.nc',
o.test_data_folder() + '16Nov2015_NorKyst_z_surface/arome_subset_16Nov2015.nc'], lazy=False)
t = o.env.get_variables_along_trajectory(variables=['x_sea_water_velocity', 'y_sea_water_velocity', 'x_wind', 'y_wind'],
lons=drifter_lons, lats=drifter_lats, times=drifter_times)
wind_drift_factor, azimuth = wind_drift_factor_from_trajectory(t)
o.seed_elements(lon=4, lat=60, number=1, time=ot.env.readers[list(ot.env.readers)[0]].start_time,
wind_drift_factor=0.033)
/opt/conda/envs/opendrift/lib/python3.11/site-packages/pyproj/crs/crs.py:1286: UserWarning: You will likely lose important projection information when converting to a PROJ string from another format. See: https://proj.org/faq.html#what-is-the-best-format-for-describing-coordinate-reference-systems
proj = self._crs.to_proj4(version=version)
/opt/conda/envs/opendrift/lib/python3.11/site-packages/pyproj/crs/crs.py:1286: UserWarning: You will likely lose important projection information when converting to a PROJ string from another format. See: https://proj.org/faq.html#what-is-the-best-format-for-describing-coordinate-reference-systems
proj = self._crs.to_proj4(version=version)
New simulation, this time without diffusivity/noise
o.run(duration=timedelta(hours=12), time_step=600)
o.plot(fast=True, legend=True, drifter=drifter)
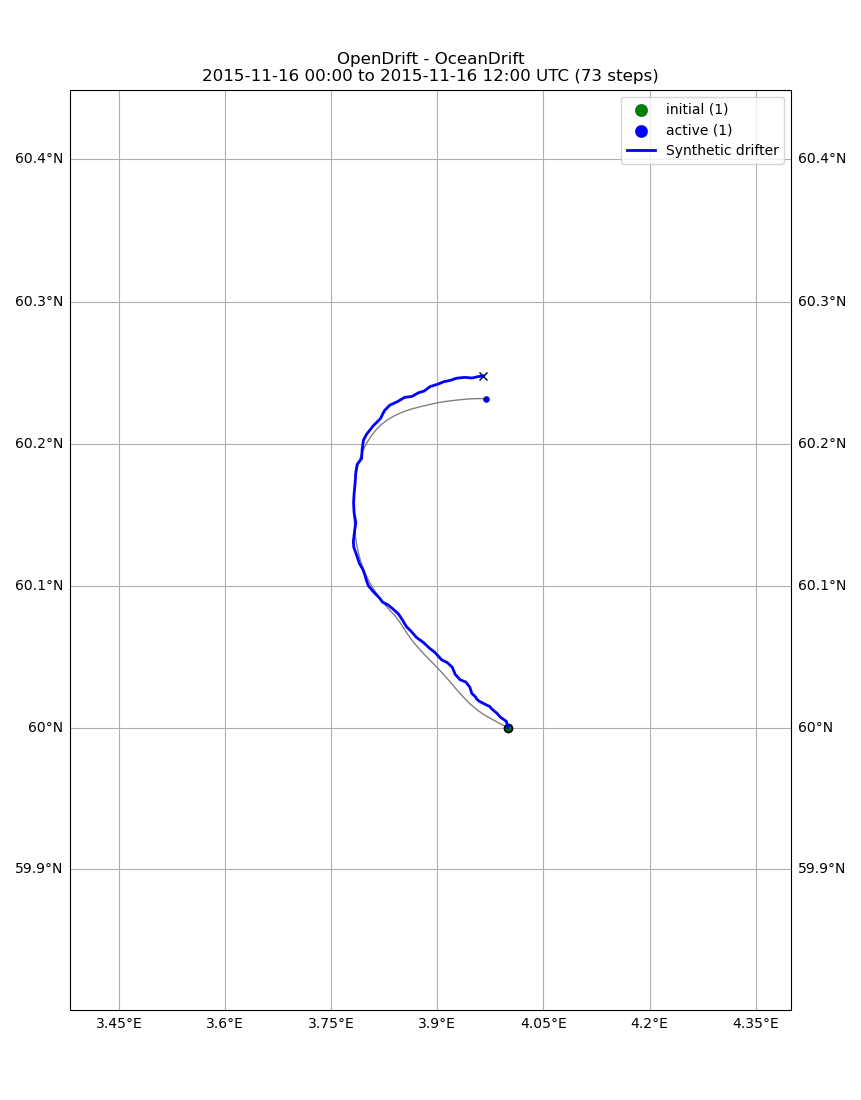
(<GeoAxes: title={'center': 'OpenDrift - OceanDrift\n2015-11-16 00:00 to 2015-11-16 12:00 UTC (73 steps)'}>, <Figure size 861.433x1100 with 1 Axes>)
The mean retrieved wind_drift_factor is 0.036, slichtly higher than the value 0.033 used for the simulation. The difference is due to the “noise” added by horizontal diffusion. Note that the retieved wind_drift_factor is linked to the wind and current used for the retrieval, other forcing datasets will yeld different value of the wind_drift_factor.
print(wind_drift_factor.mean())
plt.hist(wind_drift_factor, label='Retrieved wind_drift_factor')
plt.axvline(x=0.033, label='Actual wind_drift_factor of 0.033', color='k')
plt.axvline(x=wind_drift_factor.mean(), label='Mean retieved wind_drift_factor of %.3f' % wind_drift_factor.mean(), color='r')
plt.ylabel('Number')
plt.xlabel('Wind drift factor [fraction]')
plt.legend(loc='lower left')
plt.show()
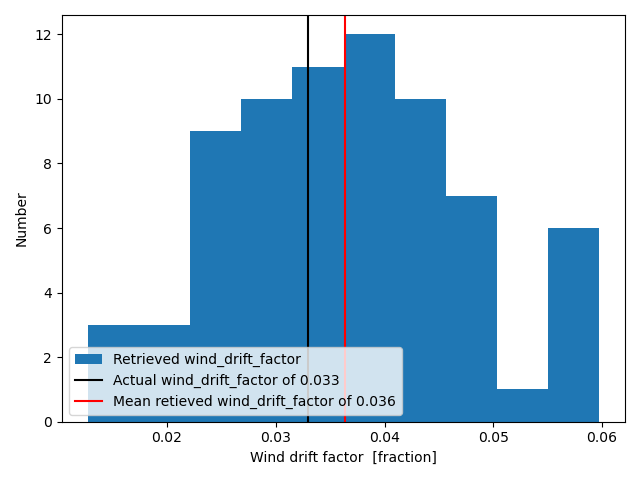
0.03639823255601244
A polar 2D histogram showing also the azimuth offset direction of the retrieved wind drift factor. See Sutherland et al. (2020), https://doi.org/10.1175/JTECH-D-20-0013.1
wmax = wind_drift_factor.max()
wbins = np.arange(0, wmax+.005, .005)
abins = np.linspace(-180, 180, 30)
hist, _, _ = np.histogram2d(azimuth, wind_drift_factor, bins=(abins, wbins))
A, W = np.meshgrid(abins, wbins)
fig, ax = plt.subplots(subplot_kw=dict(projection='polar'))
ax.set_theta_zero_location('N', offset=0)
ax.set_theta_direction(-1)
pc = ax.pcolormesh(np.radians(A), W, hist.T, cmap=cmocean.cm.dense)
plt.arrow(np.pi, wmax, 0, -wmax, width=.015, facecolor='k', zorder=100,
head_width=.8, lw=2, head_length=.005, length_includes_head=True)
plt.text(np.pi, wmax*.5, ' Wind direction', fontsize=18)
plt.grid()
plt.show()
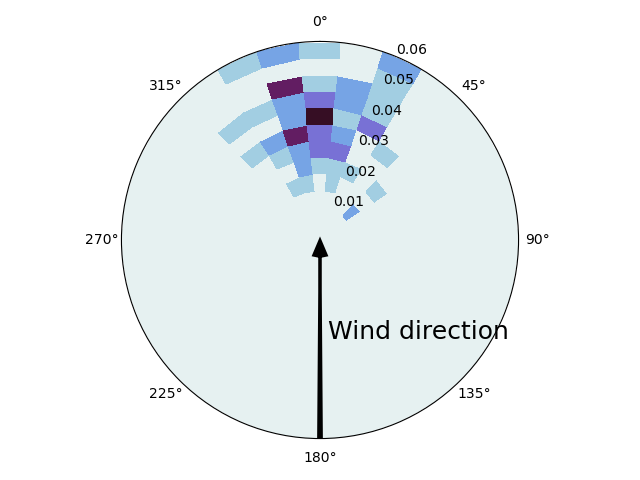
Alternative method, using skillscore
Here we calculate trajectories for a range of wind_drift_factors, and calculate the Liu-Weissberg skillscore for each. The optimized wind_drift_factor corresponds to the maximum skillscore.
o = OceanDrift(loglevel=50)
o.add_readers_from_list([o.test_data_folder() +
'16Nov2015_NorKyst_z_surface/norkyst800_subset_16Nov2015.nc',
o.test_data_folder() + '16Nov2015_NorKyst_z_surface/arome_subset_16Nov2015.nc'], lazy=False)
/opt/conda/envs/opendrift/lib/python3.11/site-packages/pyproj/crs/crs.py:1286: UserWarning: You will likely lose important projection information when converting to a PROJ string from another format. See: https://proj.org/faq.html#what-is-the-best-format-for-describing-coordinate-reference-systems
proj = self._crs.to_proj4(version=version)
/opt/conda/envs/opendrift/lib/python3.11/site-packages/pyproj/crs/crs.py:1286: UserWarning: You will likely lose important projection information when converting to a PROJ string from another format. See: https://proj.org/faq.html#what-is-the-best-format-for-describing-coordinate-reference-systems
proj = self._crs.to_proj4(version=version)
Calulating trajectories for 100 different wind_drift_factors between 0 and 0.05
wdf = np.linspace(0.0, 0.05, 100)
o.seed_elements(lon=4, lat=60, time=ot.env.readers[list(ot.env.readers)[0]].start_time,
wind_drift_factor=wdf, number=len(wdf))
o.run(duration=timedelta(hours=12), time_step=600)
o.plot(linecolor='wind_drift_factor', drifter=drifter)
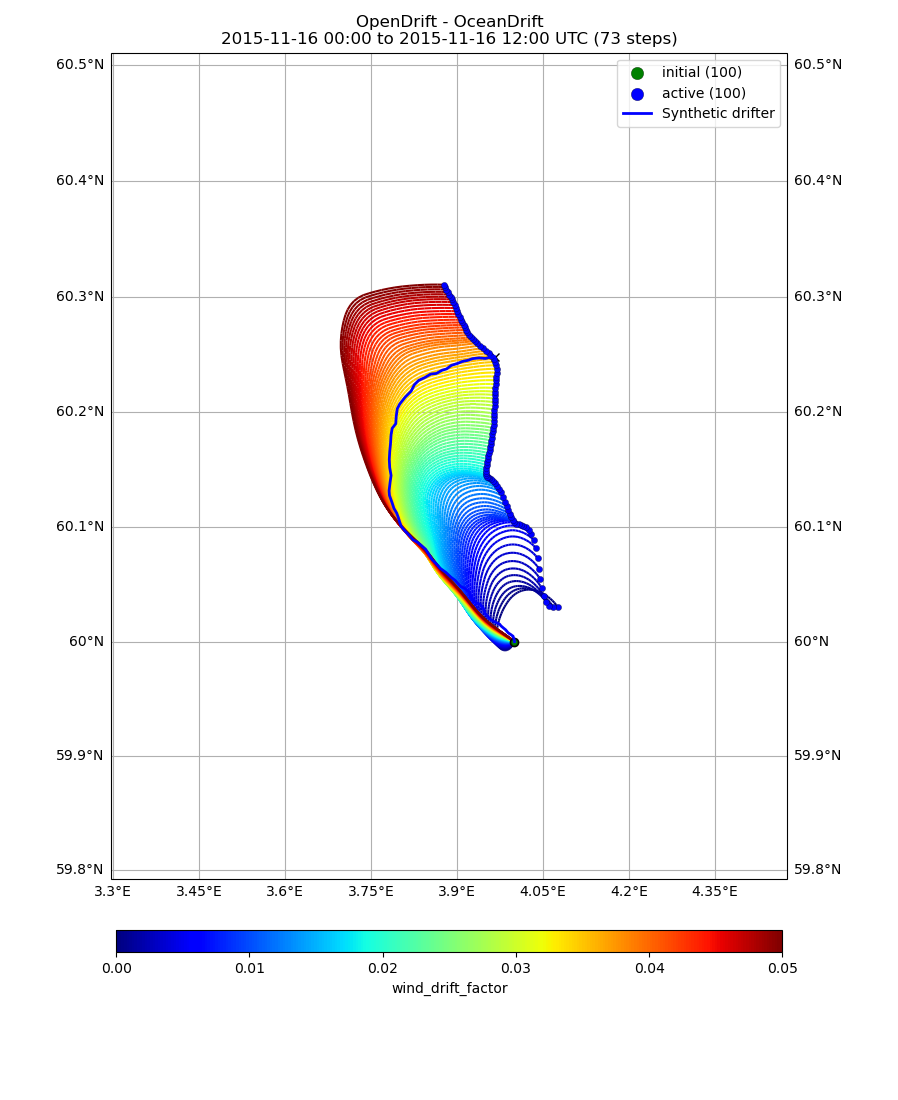
(<GeoAxes: title={'center': 'OpenDrift - OceanDrift\n2015-11-16 00:00 to 2015-11-16 12:00 UTC (73 steps)'}>, <Figure size 898.669x1100 with 2 Axes>)
Plotting and finding the wind_drift_factor which maximises the skillscore
skillscore = o.skillscore_trajectory(drifter_lons, drifter_lats, drifter_times, tolerance_threshold=1)
ind = np.argmax(skillscore)
plt.plot(wdf, skillscore)
plt.xlabel('Wind drift factor [fraction]')
plt.ylabel('Liu-Weissberg skillscore')
plt.title('Maximum skillscore %.3f for wdf=%.3f' % (skillscore[ind], wdf[ind]))
plt.show()
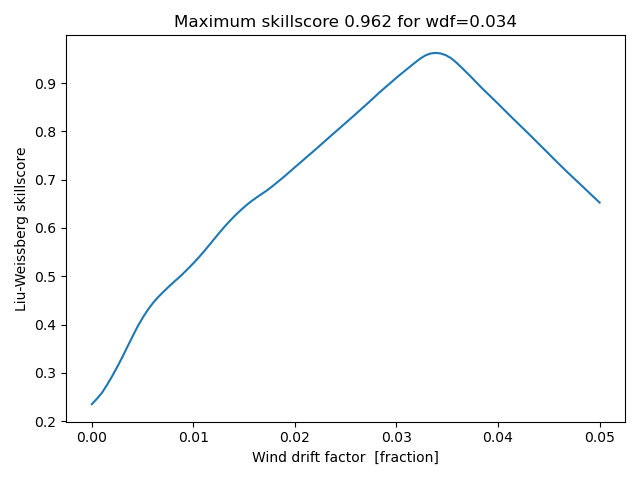
We see that using skillscore from the full trajectories gives a better estimate than calculating the average of the wind_drift_factor from one position to the next (i.e. polar histogram above). This is even more clear if increasing the diffusivity (i.e. noise) above from 10 m2/s to 200 m2/s: The histogram method then gives 0.071, which is much to high (true is 0.033), and the histogram is noisy. The skillscore method is still robust, and gives a wind_drift_factor of 0.036, only slightly too high.
Total running time of the script: (0 minutes 48.869 seconds)